What is Adversarial Machine Learning?
Adversarial Machine Learning (AML)
Adversarial Machine Learning (AML), an emerging field in the machine learning landscape, has created waves due to its unique characteristics and complexity. Utilizing techniques and concepts from both machine learning and cybersecurity, adversarial machine learning facilitates machine learning models in more hostile environments and under potential threats.
Characteristics of Adversarial Machine Learning:
• Diverse Applicability: AML has found extensive uses across multiple industry niches, from cybersecurity to autonomous vehicles, medical diagnostics, and even social media networks due to its unique capabilities.
• Robust Model: It fosters developing robust machine learning models which can detect and resist any manipulations or attacks, thus ensuring the model's efficiency and reliability.
• Resilience: Models are regularly tested under various adversarial situations to determine their ability to bounce back or withstand potential threats or attacks, thereby increasing their overall resilience.
• Advanced Security: Adversarial ML takes machine learning to the next level by incorporating advanced security measures to protect ML models.
• Finite Adaptation: Adaptation and modifications to the ML models are carefully employed to maintain the stability and authenticity of the models.
Implementing Adversarial Machine Learning
While planning the implementation of adversarial machine learning, thorough understanding of the organization's needs, resources, and potential threats is required. Emphasizing evaluating and selecting the most suitable adversarial technique, assessing costs linked with resources, and ensuring the seamless integration with existing frameworks will aid in ensuring that adversarial machine learning enhances the robustness and efficiency of ML models. In turn, regular monitoring and reviews can contribute to keeping the entire system updated and resilient in the face of ever-evolving threats.
Artificial Intelligence Master Class
Exponential Opportunities. Existential Risks. Master the AI-Driven Future.
Pros of Adversarial Machine Learning
AML, while a complex field, offers a compelling set of advantages:
• Enhanced Security: Since security is a prime concern in the digital world, the fundamental appeal of AML is its contribution towards enhancing the security framework of different ML models by assessing them in manipulated environments.
• Preparedness: By creating adversarial scenarios, the robustness of machine learning models is tested, thus sharpening the preparedness of models for potential threats.
• Resourceful: Using AML, organizations can save valuable resources by training their existing ML models to maintain efficiency in the face of potential threats.
• Versatility: It enhances the versatility of ML models by preparing them for different hostile environments, increasing their applicability and efficiency.
Cons of Adversarial Machine Learning
Despite its potential advantages, AML presents some concerns, including:
• Complex Implementation: The implementation of adversarial attacks for testing ML models requires a comprehensive understanding of both ML and cybersecurity, making the process intricate and complex.
• Increased Dependence: Organizations might become overly reliant on AML, thus increasing their vulnerability if the method is used dishonorably.
• Limited Scalability: The robustness of the adversarial environment might limit the scalability of ML models in various situations.
• Intense Resource Requirements: The process of adversarial learning often requires the investment of significant computational resources, thereby raising cost-related concerns.
• Increased Risk: The development and use of adversarial machine learning techniques can potentially aid malicious attackers in identifying and exploiting vulnerabilities in ML models.
• Potential for Overfitting: There is always a risk that too much emphasis on building robustness to adversarial attacks could cause the model to be overfit to the training data and perform poorly on the real-world data.
Understanding the benefits and limitations of adversarial machine learning is pivotal for organizations seeking to implement it in their operations. Careful assessment of the specific circumstances, availability of resources, and expert knowledge can drive the successful integration of AML in an organization’s ecosystem.
Take Action
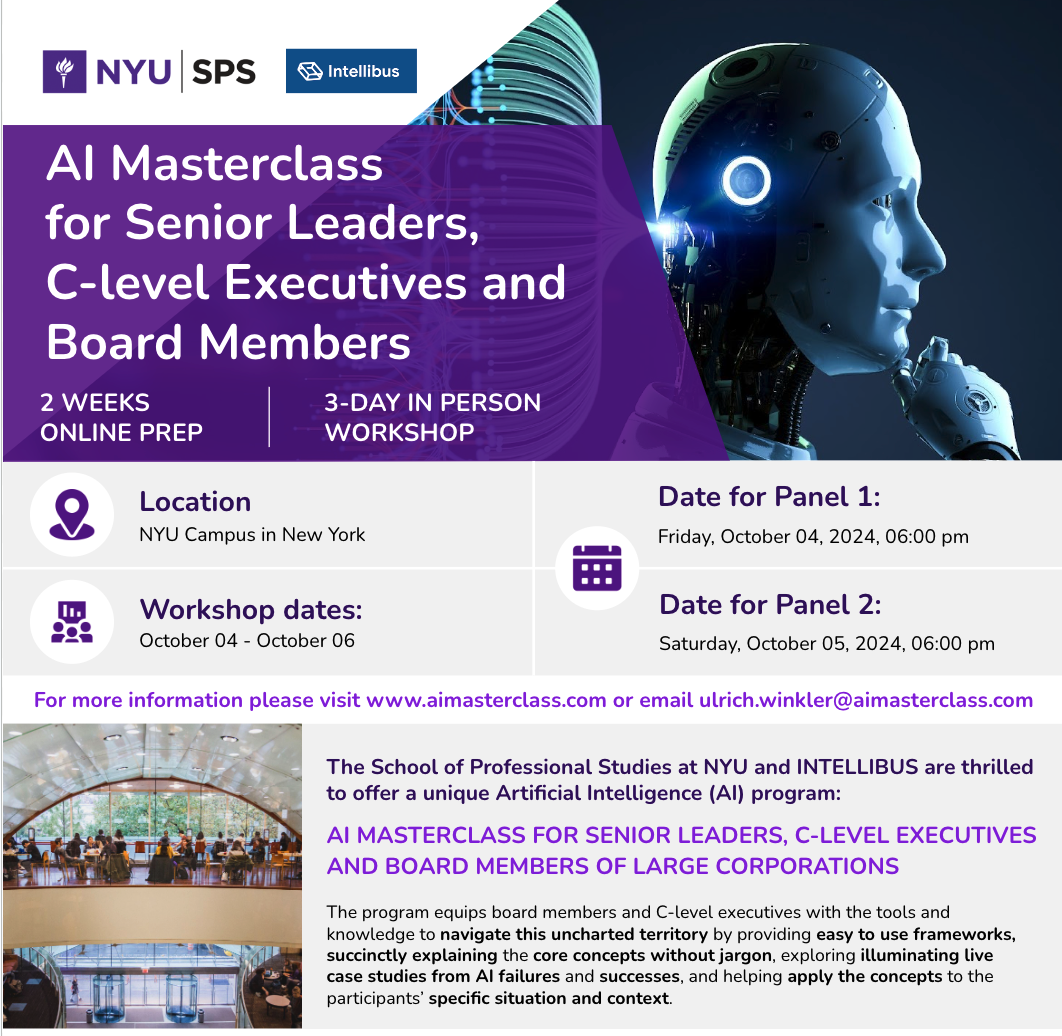
Download Brochure
- Course overview
- Learning journey
- Learning methodology
- Faculty
- Panel members
- Benefits of the program to you and your organization
- Admissions
- Schedule and tuition
- Location and logistics