What is Adversarial Training for Robustness?
Adversarial Training for Robustness: An In-depth Analysis
Adversarial training, a technique commonly utilized in the world of machine learning, particularly in the development and enhancement of artificial intelligence models, entails the creation of adversarial examples to improve overall security and model performance. Adversarial examples are typically crafted to mislead AI models, subsequently bending the model's output in a predetermined direction. The robustness of machine learning models gains significantly through adversarial training, which teaches the models to identify and defend against such adversarial attacks.
Key characteristics of Adversarial Training:
Resilience Enhancement: Adversarial training strengthens models against adversarial attacks by helping them recognize and resist the deceptive data points that adversaries create.
Performance Improvement: The approach contributes to an AI model's overall performance enhancement, as training with adversarial examples often results in improved generalization capabilities.
Advanced Security: The ability to withstand adversarial attacks results in a secure AI model, safe from malevolent entities seeking to exploit model vulnerabilities.
Real-World Applicability: Adversarial training finds use in diverse applications due to its potential in various industries, particularly those relying heavily on deep learning models.
Automated Learning: It forms the core of the model's capability to automate its self-improvement, learning from each experience to resist future adversarial attacks.
Artificial Intelligence Master Class
Exponential Opportunities. Existential Risks. Master the AI-Driven Future.
Advantages and Downsides of Adversarial Training
Apart from its core role enhancing AI model resilience, adversarial training can also offer several benefits:
Robustness: Adversarial training effectively increases model robustness against adversarial attacks, reducing the likelihood of wrong predictions or outputs when exposed to adversarial examples.
Improved Accuracy: Training with adversarial examples can effectively improve model prediction accuracy and performance, even with non-adversarial examples.
Enhanced Security: By making the model resistant to adversarial attacks, adversarial training boosts the model's overall security, shielding it from potential intrusion attempts.
- Wide-Range Application: Adversarial training demonstrates versatility, applicable in diverse fields including healthcare, finance, and transportation, among others.
However, despite these impressive benefits, adversarial training also presents certain challenges:
Relatively High Computational Cost: Adversarial training may require higher computational resources than standard model training, due to the need for generating adversarial attacks and retraining the model repeatedly.
Risk of Overfitting: Overreliance on adversarial training may lead to overfitting, where the model becomes excessively specialized in the training data, diminishing its performance with unseen, real-world data.
Potential Security Weaknesses: While the approach effectively counters known adversarial attacks, novel, unseen adversarial techniques could potentially exploit the model.
Adversarial training implementation necessitates a meticulous approach, requiring a thorough understanding of adversarial attacks and an appropriate strategy to generate adversarial examples effectively. It also emphasizes the need for periodic reviews and updates to maintain model robustness against emerging threats.
A proactive adversarial training implementation involves comprehensive planning and strategizing, setting up suitable infrastructure and resources, and optimizing the training process to ensure maximum model robustness. Monitoring and updating the process form a critical aspect of this implementation, securing the trained model against evolving adversarial methods. In summary, a well-crafted adversarial training system based on organizational needs, opportunities, and awareness of potential challenges, engenders robust, well-performing AI models, playing a crucial role in harnessing the full potentials of machine learning technologies.
Take Action
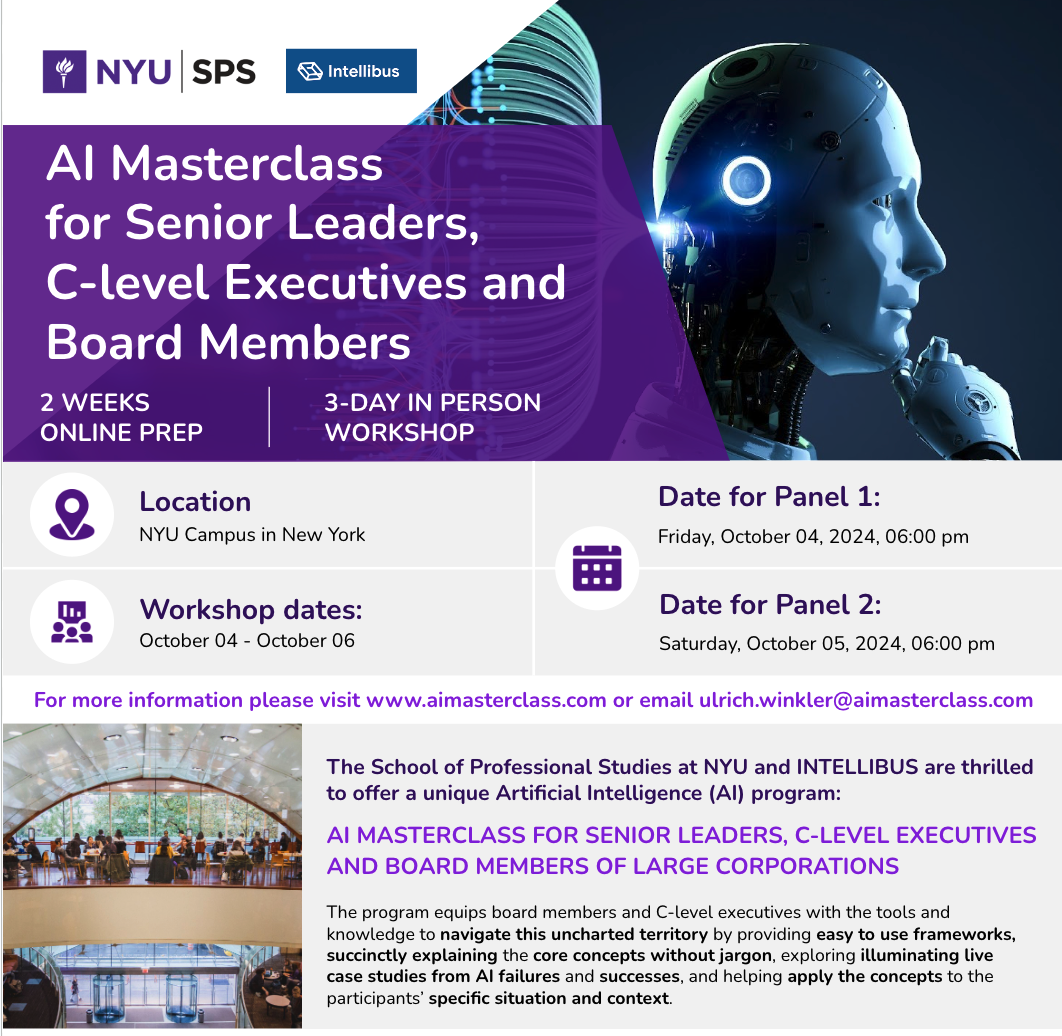
Download Brochure
- Course overview
- Learning journey
- Learning methodology
- Faculty
- Panel members
- Benefits of the program to you and your organization
- Admissions
- Schedule and tuition
- Location and logistics