What is Agent-Based Modeling?
Understanding Agent-Based Modeling
Agent-Based Modeling, also known as ABM, deals with a computational model utilized for simulating the actions and interactions of autonomous entities in a network. These entities, referred to as 'agents,' aim to decipher how the actions of individual components can affect the whole. ABM software systems have a broad application scope and are specifically designed to cater to different sectors' requirements, from economics to social sciences, and from biology to computer science.
ABM Software exhibits some key characteristics:
Simplicity and Flexibility: With ABM, one can adopt 'simple rules' to examine 'complex behaviors.' Unlike traditional modeling techniques, ABM offers flexibility- enabling the modeling of complex systems with multiple interactions.
Decentralized Approach: The agents in the ABM methodology receive programming to act independently as per a set of rules. This approach enables the simulation of complex systems through the interactions of these individual, mutual components.
Adaptive Agents: In ABM, the agents are usually adaptive, meaning they can learn from their experiences and adjust their behavior accordingly, contributing to the model's dynamics.
- Local Interactions: ABM usually allows for localized interactions among agents, which can result in system-level patterns.
ABM applications are prevalent in sectors that involve complex systems with decentralized, interacting parts, which can benefit from the study of system-level emergence based on individual components' actions.
Artificial Intelligence Master Class
Exponential Opportunities. Existential Risks. Master the AI-Driven Future.
Advantages and Downsides of ABM
Let’s delve into some inherent advantages associated with this computational approach:
Micro-Level Insights: Details are critical in ABM as agents can represent anything- individuals, groups, or organizations. Thus, it allows for micro-level examination and understanding.
Real-World Simulation: ABM assists in realistically simulating and understanding complex systems by integrating various components and their interdependencies.
Flexibility: ABM offers flexibility and can model systems with multiple complex interactions, which traditional modeling techniques often find challenging to handle.
- Visualized Process: ABM provides a visual way of representing complex systems, assisting in conceptual understanding and interpretation.
ABM effectively handles non-linearity, feedback loops, and emergent phenomena, making it an indispensable tool for understanding and predicting complex systems' behaviors.
Notwithstanding its advantages, there are potential challenges with the ABM software:
Computational Intensity: Since ABM involves a multitude of agents interacting simultaneously, it can get computationally demanding and resource-intensive.
Limited Scalability: While one can represent many agents in an ABM model, managing these agents' rules and interactions proves challenging as their number increases. Thus, it imposes limitations on the model's scalability.
Model Validation: As ABM deals with complex systems and stochastic behaviors, validating these models can be challenging, and their predictive accuracy might not be precise.
Despite these challenges, with careful planning and robust computational resources, the potential of Agent-Based Modeling in understanding complex systems remains significant.
Implementation of ABM
The implementation of an ABM approach necessitates a comprehensive understanding of the system under study and the agents' precise definition and the rules governing their behavior. Additionally, a thorough examination of the model's scope, the level of detail required, and the computational resources available is also mandatory. Once this groundwork is laid, the process moves on to the actual modeling stage – involving setting up the environment, defining the agents and their behavior, and implementing the interactions. Ultimately, the model's outcomes are analyzed and validated to ensure an accurate, representative simulation of the complex system.
In sum, while Agent-Based Modeling might involve complex planning and intensive resources, its capacity to simulate and dissect complex systems' behavior gives it an unparalleled advantage. Thus, making it an essential tool for studying complex systems in various fields.
Take Action
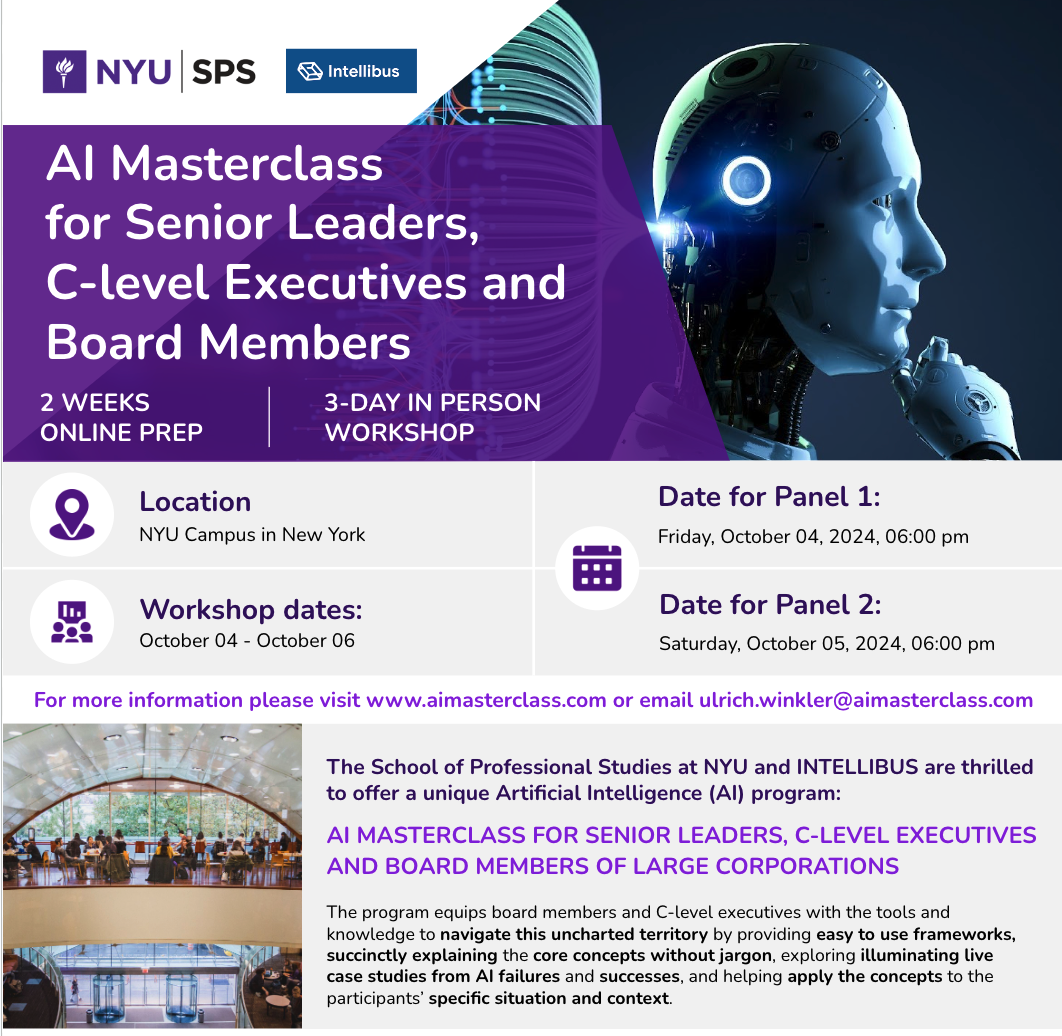
Download Brochure
- Course overview
- Learning journey
- Learning methodology
- Faculty
- Panel members
- Benefits of the program to you and your organization
- Admissions
- Schedule and tuition
- Location and logistics