What is Bayesian Inference Methods?
What is Bayesian Inference?
In the world of statistics, the Bayesian method, commonly known as Bayesian Inference, is a method of inference that is heavily grounded in the principles of probability. Bayesian inference is aptly named after Thomas Bayes, an 18th century statistician and mathematician known for his work on probability theory. The value of Bayesian Inference lies in its ability to provide a mathematical blueprint to deal with complex systems, update information based on evidence and deal with uncertainty within complex data sets.
Distinctive Characteristics of Bayesian Inference:
- Flexibility: Bayesian inference allows for flexibility in modeling data. It has the capacity to deal with a wide range of complex data situations and structures that classical statistical methods often struggle to address.
- Uncertainty Quantification: Bayesian inference allows for the quantification of uncertainty. As models are updated with new data, the uncertainty in the parameter estimates decreases.
- Parameter Estimation: Bayesian models yield full probabilistic model parameters. Bayesian inference can provide estimates for all parameters included in the model.
- Prior Knowledge Incorporation: Bayesian inference allows for the integration of previous knowledge (prior) with observed data (likelihood) to make probabilistic statements about unknown quantities (posterior).
- Model Complexity Handling: Unlike classical methods that become complicated with many variables, Bayesian models can accommodate a wide range of complexities attributed to the hierarchical structures used in these models.
Usage of Bayesian Inference
Bayesian inference has extensive applications in various sectors, from medical research to finance to machine learning algorithms. The value of Bayesian inference lies in its flexibility and ability to provide probabilistic statements that explain uncertainty.
Implementation of Bayesian Inference
Implementing Bayesian inference necessitates robust programming skills and a profound understanding of probability theory. Modern software packages, like Stan, PyMC3, JAGS, or BUGS, have made the implementation of Bayesian models more accessible. Nevertheless, careful model building and checking, as well as a thorough understanding of the underlying theory, are crucial to successful Bayesian analysis and interpretation. The Bayesian approach, with its emphasis on learning from data and quantifying uncertainty, embodies the scientific method and suits the way human beings encounter and learn about the world.
Artificial Intelligence Master Class
Exponential Opportunities. Existential Risks. Master the AI-Driven Future.
Advantages of Bayesian Inference
There are several benefits to Bayesian inference, among them include:
- Prior Knowledge Integration: Bayesian inference permits the incorporation of past evidence into ongoing data analysis - ultimately delivering more precise and insightful predictions.
- Dynamic Updates: With Bayesian inference, the approach towards analyzing data is dynamic. As fresh data becomes available, models can be updated, allowing for real-time decisions based on the most recent data.
- Comprehensive Predictive Distribution: Bayesian models give a complete distribution of predictions, which allows for better risk management in decision making.
- Model Uncertainty: Bayesian inference can handle large complex models by utilizing the posterior predictive distribution to include both parameter uncertainty and model uncertainty.
- Complex Data Structures Handling: Bayesian inference excels at handling and analyzing complex data structures that may be too intricate for traditional statistical methods.
Disadvantages of Bayesian Inference
While there are many advantages to using Bayesian inference, there are also downsides:
- Computational Intensity: Bayesian models are typically more computationally intensive than frequentist ones. MCMC sampling can take a long time, especially for large datasets or complex models.
- Dependency on Prior: The selection of an appropriate prior can be a challenging and subjective process. Inappropriate choice of prior can influence the inferences.
- Lack of Widespread Understanding: Despite its theoretical grounding, Bayesian inference is not universally understood in the same manner as traditional statistical methods are.
Take Action
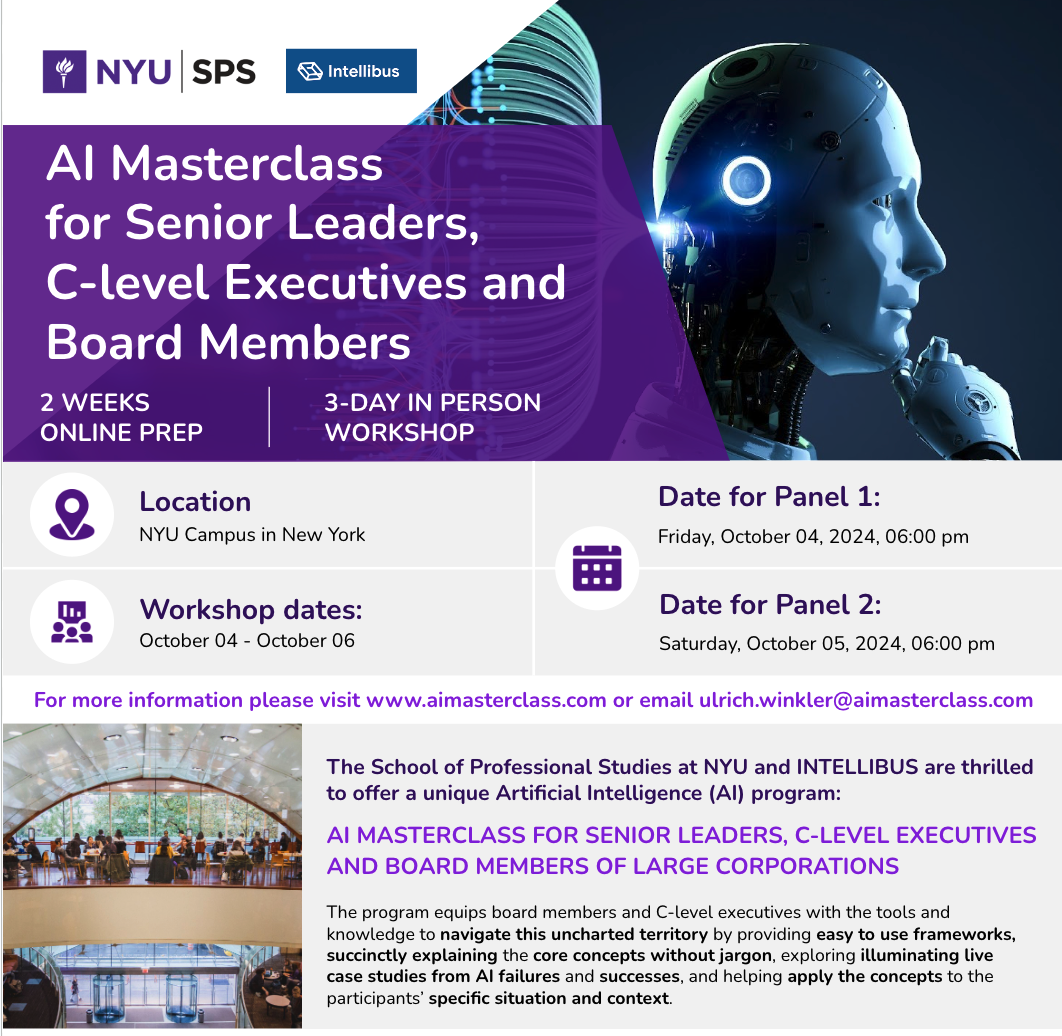
Download Brochure
- Course overview
- Learning journey
- Learning methodology
- Faculty
- Panel members
- Benefits of the program to you and your organization
- Admissions
- Schedule and tuition
- Location and logistics