What is Causal Inference Models?
Understanding Causal Inference Models: A Detailed Examination
Causal inference models, or simply causal models, form the backbone of a methodological framework that allows analysts to discern cause-and-effect relations based on observational data. Broadly speaking, these models are designed to analyze the effects of a treatment, policy, or an event (collectively termed “interventions”) on an outcome of interest.
The utility of causal inference models is far-reaching; they are employed in numerous disciplines, such as economics, political science, and epidemiology, and are crucial for decision-making and policy planning, as well as forecasting and effect-calculation in a wide array of contexts.
One can classify these models based on the fundamental characteristics they exhibit:
- Wide Accessibility: These models are readily available to researchers and analysts via statistical software or can be developed in-house,
- Standard Functionality: They typically encompass sophisticated methodologies designed to control for confounders and account for selection bias, thereby enabling robust causal effect estimation.
- Minimal Customization: Although customization is possible in the sense of selecting different model specifications, the underlying principles of inference from causality usually remain consistent across applications.
- Community Support: Regular updates, academic discussions, and software patches are often available, ensuring the models' relevance and effectiveness over time.
- Cost-Effectiveness: Owing to their wide availability in statistical software, these models are generally cost-effective alternatives to designing proprietary models from scratch.
Implementation of Causal Inference Models
To maximize the utility of these models, it's vital to conduct a thorough analysis of the research question at hand, followed by a careful selection of the suitable model specification. Appropriate training data is then used to fine-tune the model, after which it can be deployed. It’s worth noting that a firm understanding of the assumptions and statistical properties underlying these models, as well as the nuances of the particular application, is vital to ensure their effective utilization.
Artificial Intelligence Master Class
Exponential Opportunities. Existential Risks. Master the AI-Driven Future.
Advantages of Causal Inference Models
Organizations and researchers often rely on causal inference models thanks to their numerous advantages:
- Cost-Effectiveness: As stated earlier, these models are typically more affordable than developing proprietary models from the ground up.
- Reduced Development Time: Since causal inference models are readily available, they considerably reduce the time and effort of developing a model from scratch.
- Proven Reliability: These models have been used extensively in varied disciplines and have been validated across a wide range of scenarios.
- Regular Updates and Support: Causal models used off-the-shelf from statistical packages benefit from the regular updates and patches provided by the software vendors.
- Versatility and Compatibility: Causal inference models can be applied to a diversity of applications and are usually adaptable and compatible across a range of statistical software.
- Standards Compliance: These models align with methodological and statistical standards, aiding in smoother reporting and validating of outcomes.
Disadvantages of Causal Inference Models
Despite their numerous advantages, there are certain limitations to consider:
- Limited Customization: Given that these models are designed to cater to a wide array of applications, they may not always align perfectly with a specific experimental setup or research question.
- Dependence: Reliance on a set of assumptions inherent in causal models can sometimes limit their application.
- Accuracy Constraints: As causal relationships cannot be directly observed, estimates derived from these models come with a degree of uncertainty.
- Hidden Costs: Though the direct cost of adopting these models could be low, there may be associated indirect costs (e.g., for data preparation, software licensing, and training).
In conclusion, causal inference models are powerful tools in the field of analytics and research. Although they have their limitations, these models continue to provide actionable insights across various fields, thereby aiding in decision-making, policy formation, and prediction tasks. Despite some inherent challenges, the strengths of causal inference models certainly outweigh potential drawbacks, making them an indispensable part of the modern analytical toolkit.
Take Action
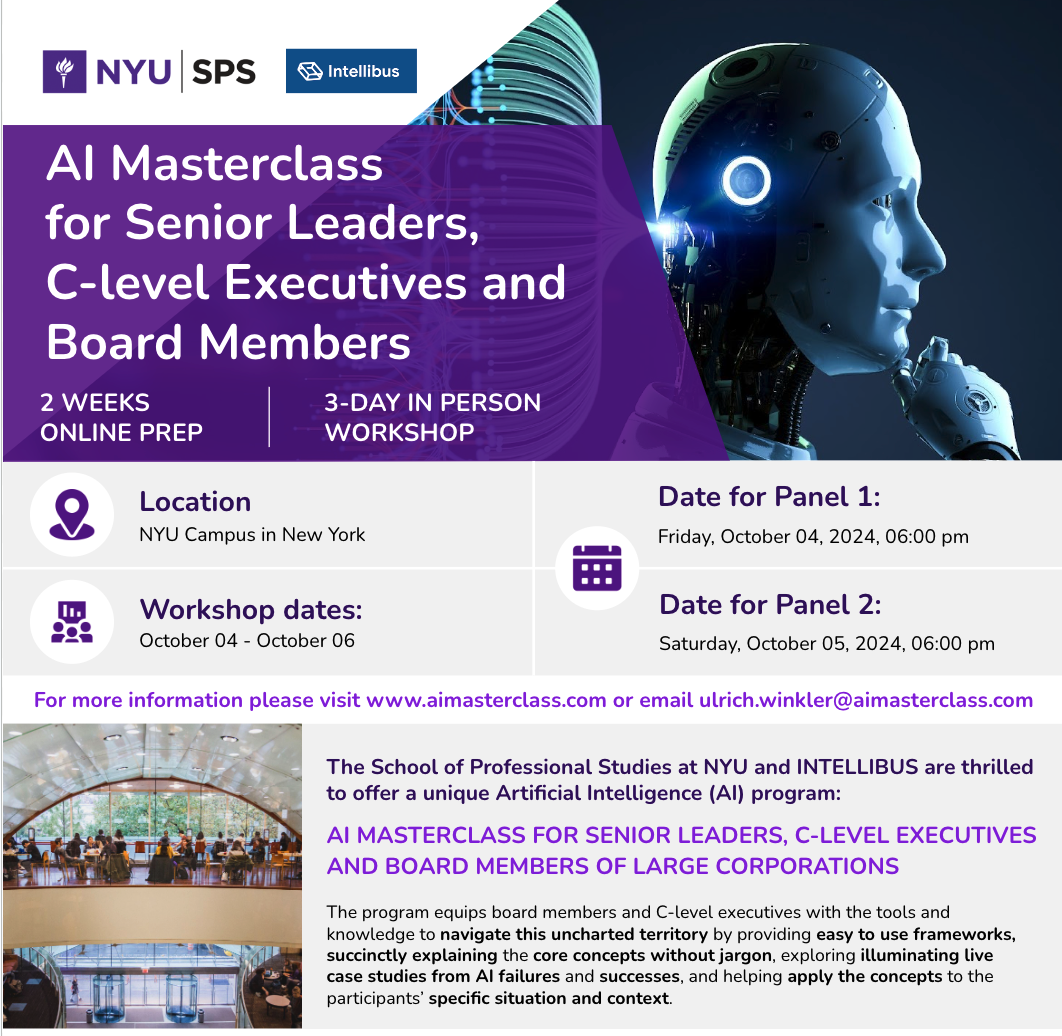
Download Brochure
- Course overview
- Learning journey
- Learning methodology
- Faculty
- Panel members
- Benefits of the program to you and your organization
- Admissions
- Schedule and tuition
- Location and logistics