What is Cross-Modal Learning in AI?
Cross-Modal Learning in Artificial Intelligence
Cross-modal learning or cross-modal transfer (CMT) represents a unique concept in the realm of artificial intelligence (AI). It leverages information from one modality to improve performance in another, thus creating a versatile learning model. For instance, the concept of CMT can allow an AI model to learn from visual data and apply the gleaned knowledge to auditory data tasks, or vice versa.
Key Features of Cross-Modal Learning in AI
Cross-Modal Learning in AI boasts several specific features which include:
- Multidimensional Learning: Cross-modal learning acknowledges the vast dimensions of data. It allows an AI model to comprehend and learn from multiple modalities of data such as images, text, and audio.
- Improved Performance: Employing cross-modal learning can enhance the performance of an AI model as it can leverage diverse data types to bolster learning and improve its prediction accuracy.
- Data Enrichment: Cross-modal learning facilitates data enrichment since AI models can benefit from the interplay between different data modalities.
- Increased Flexibility: Cross-modal learning renders AI more flexible as it allows the exploration of insights gathered from one modality to inform understanding in another, even when original modality data isn't available.
Implementation of Cross-Modal Learning in AI
Implementing cross-modal learning in AI requires strategic planning, considering the organizations' existing data capabilities and resources. The suitability of this approach has to be meticulously assessed by understanding the advantages it can offer and the obstacles that may arise. Given the unique demands of coordinating multiple data types, it is crucial to ensure the right computational infrastructure is in place, along with strict data privacy and security protocols.
Once the decision is made, careful development and training of the cross-modal learning model are crucial to ensure optimal results. This may necessitate ongoing iterations and a substantial investment in the process. Considering these factors, deploying cross-modal learning in AI should be conducted with a clear understanding of the organizational needs and available resources.
Artificial Intelligence Master Class
Exponential Opportunities. Existential Risks. Master the AI-Driven Future.
Advantages of Cross Modal Learning in AI
One can ascribe numerous benefits to the utensilization of cross-modal learning in Artificial Intelligence, such as:
- Performance Enhancement: Employing various modalities of data during the training phase helps the model to learn more about tasks, leading to an improvement in performance.
- Data Usage Maximization: Cross-modal learning leverages all available data from different modalities, thus ensuring data usage is maximized for better learning outcomes.
- Flexibility: The effective interaction between differing modalities promotes more flexibility in AI models since insights from one can be used to inform another, broadening their learning scope.
- Robust Decision-Making: The interface of varying data modalities makes AI models more robust to noise or inaccurate data points, as they can tap into multiple sources for data confirmation, leading to more informed decision-making.
Disadvantages of Cross-Modal Learning in AI:
Despite offering several benefits, organizations must consider the following challenges of cross-modal learning:
- Data Misalignment: Coordinating multiple data modalities can be challenging, as misalignments may occur leading to accuracy reduction.
- Computational Overhead: Processing and coordinating varying data types can add a computational overhead which could impact the performance of AI models.
- Privacy Issues: Cross-modal learning uses data from several sources, potentially raising privacy concerns as personal information from different modalities could be combined.
- Deployment Complexity: The deployment of cross-modal learning models can be complex due to the tech infrastructure required to process, coordinate, and analyze multiple data modalities seamlessly.
In closing, cross-modal learning in AI opens up a new frontier of possibilities for AI models, providing them with the ability to leverage information across modalities. Implementing this approach requires in-depth planning and investment. Despite the challenges, the potential offered by this method could revolutionize the future of AI performance by creating models that are more robust, flexible, and reliable.
Take Action
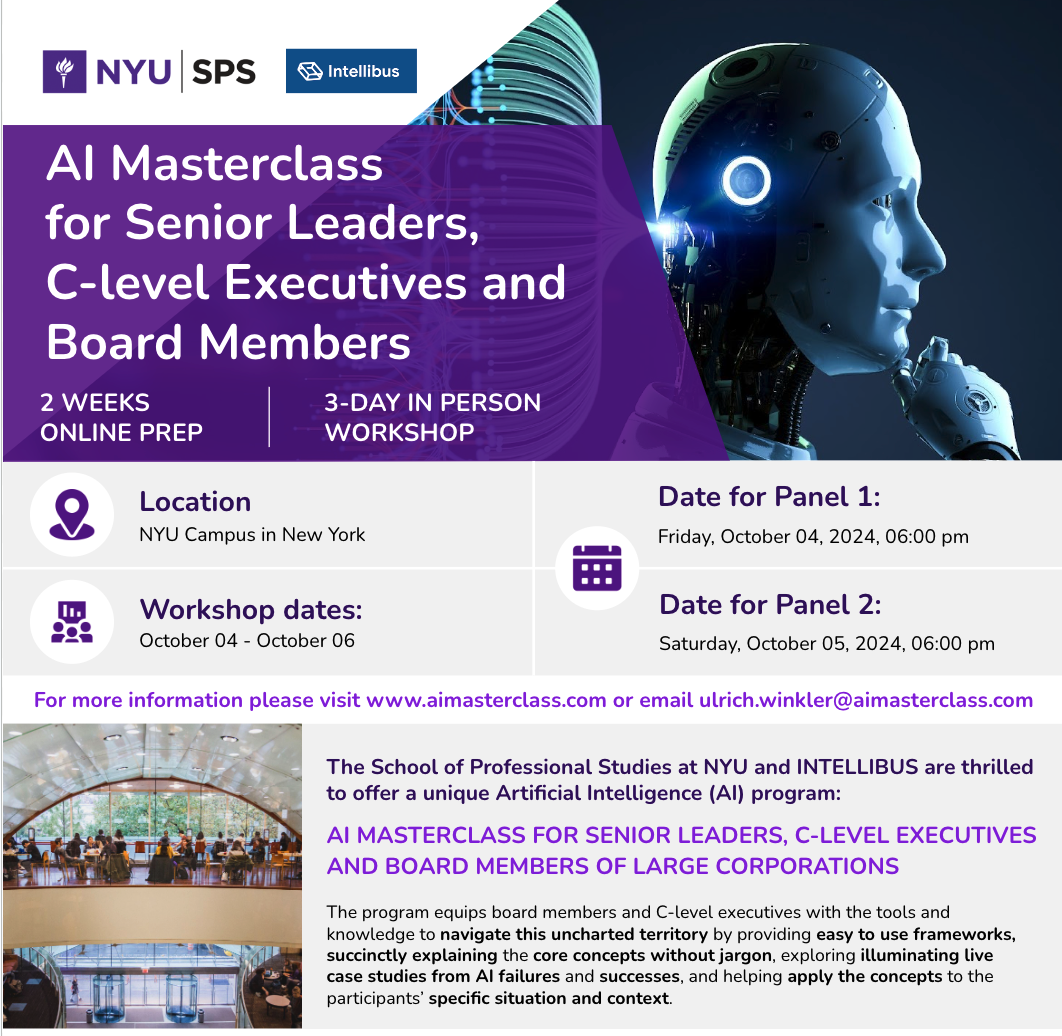
Download Brochure
- Course overview
- Learning journey
- Learning methodology
- Faculty
- Panel members
- Benefits of the program to you and your organization
- Admissions
- Schedule and tuition
- Location and logistics