What is Data Mining Techniques?
What are Data Mining Techniques?
Data mining techniques are methods used to extract high-quality, actionable information from large datasets. As the quantity of data collected and stored continues to rise, data mining techniques become more sophisticated and valuable in aiding decision making and predicting outcomes. The aim of such techniques is to enable users to navigate through the vast amount of data to discover hidden patterns and relationships, which can then be used to make informed business decisions.
Key Characteristics of Data Mining Techniques:
- Comprehensive Data Exploration: Data mining techniques sift through massive amounts of data, from various sources, in search of meaningful information.
- Predictive Modelling: They analyse current and historical data to predict future outcomes.
- Classification: These techniques are able to classify data into different categories to enhance understanding.
- Pattern Recognition: Techniques such as cluster analysis help identify patterns or correlations in data.
- Association Rule Learning: This identifies associations or connections between data items, often used in market basket analysis.
- Unsupervised and Supervised Learning: These techniques are categorized based on human intervention or lack thereof; supervised learning guides the analysis while unsupervised allows the algorithm to explore independently.
Implementing Data Mining Techniques
The implementation of data mining techniques involves a series of steps commencing with understanding business objectives and ending with model deployment. In between, data are collected, cleaned, transformed and explored, following which, a suitable model is selected and data mining performed, after which the model is evaluated, fine-tuned, and finally deployed.
- Understanding: The problem needs to be clearly understood and defined.
- Data Collection: Relevant data are collected from various sources.
- Cleaning: The data are cleaned to remove noise and inconsistencies.
- Transforming: Transformation is carried out to convert data to the appropriate forms of mining.
- Model Selection: Based on objectives, a suitable data mining method is selected.
- Data Mining: The selected method is applied to the data.
- Evaluation: The results are evaluated to ensure fulfilment of objectives.
- Fine-Tuning: If necessary, changes are made to improve the model.
- Deployment: The model is deployed to generate insights.
Data mining techniques, with their potential to unlock the valuable information within data, are already widely used in various domains. As the pace of data generation accelerates, these techniques will only become more vital and more sophisticated in the years to come. Despite some challenges, the benefits of data mining techniques far outweigh them. By understanding the processes and techniques of data mining, businesses can leverage their data effectively to gain new insights and make informed decisions. In the process, they can increase efficiency, reduce costs, and gain a significant edge over their competitors.
Artificial Intelligence Master Class
Exponential Opportunities. Existential Risks. Master the AI-Driven Future.
Benefits of Data Mining Techniques
- Cost-Effective: Unlike traditional market research methods. Data mining employs existing data, reducing the cost of gathering information.
- Efficient: Automated models sift through extensive datasets in less time than manual methods.
- Predictive Analysis: They provide accurate forecasts of future trends and behaviours.
- Strategic Decision Making: The insights enable management to make informed decisions.
- Increased Sales: They facilitate customer segmentation and targeted advertising, leading to increased sales.
- Reduced Risks: Fraud detection and risk management are enhanced.
Challenges of Data Mining Techniques
- Complexity of Data: The sheer volume and diversity of data can be daunting.
- Risk of Overfitting: The model may learn from noise in the data and not generalize well.
- Data Quality: Poor quality data will lead to poor results.
- Privacy and Security: The compilation of data may raise issues around privacy.
Data Mining Techniques in Practice
Data mining techniques are widely used across many industries due to the valuable insights they provide. They play a crucial role in business intelligence and data-driven decision making, with applications ranging from customer segmentation in marketing to fraud detection in finance, stock market analysis, healthcare, sports, and many more.
Take Action
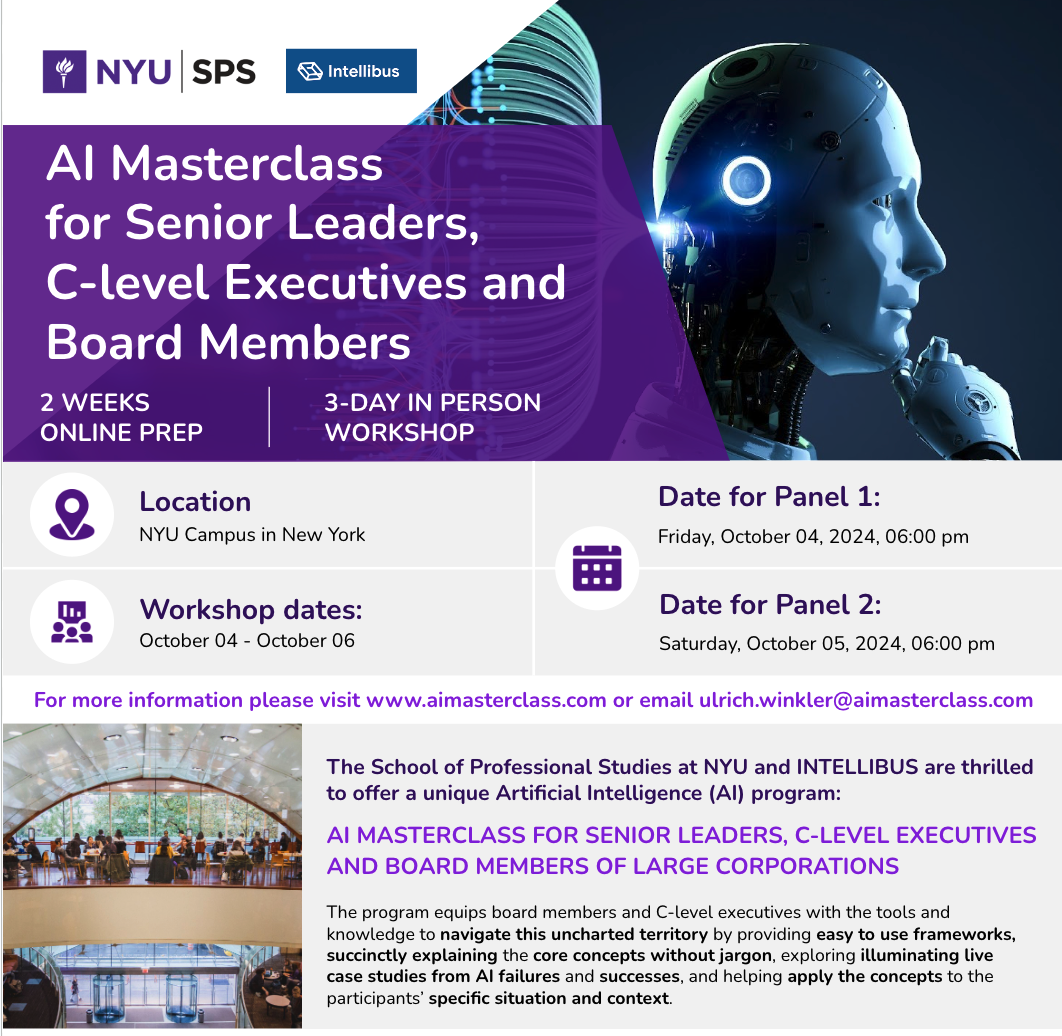
Download Brochure
- Course overview
- Learning journey
- Learning methodology
- Faculty
- Panel members
- Benefits of the program to you and your organization
- Admissions
- Schedule and tuition
- Location and logistics