What is Data Privacy in Machine Learning?
What is Data Privacy in Machine Learning?
Data privacy in machine learning stands as a fundamental element in the age of artificial intelligence, where massive volumes of data are steadily processed for decision-making and prediction purposes. This data often contains sensitive, personally identifiable information, which gives rise to serious privacy concerns. To address these concerns, machine learning must adhere to specific guidelines to protect individual data while fulfilling its function of predicting outcomes accurately.
Let's look at the key facets of data privacy in machine learning:
- Limited Information Disclosure: Machine learning models, especially those employed in public domains, should limit individual information disclosure in the results or data visualizations. This ensures that personal information is protected while providing the necessary insights.
- Anonymization of Data: Sensitive datasets for training machine learning models should, in ideal scenarios, be stripped of identifiable information to uphold privacy. This process is known as anonymization and is crucial to prevent the misuse or abuse of sensitive information.
- Secure Cloud Storage: Storing machine learning models and the corresponding datasets securely is pivotal; typically, information is cloud-stored to fortify security and promote accessibility.
- Regulatory Compliance: Machine learning, when handling data, must comply with existing privacy regulations like the General Data Protection Regulation (GDPR).
Implementation of Data Privacy in Machine Learning
Dependable data privacy in machine learning requires careful planning and execution. Considerations include understanding the data, who has access to it, and how it moves through different stages of processing. Cryptography techniques, federated learning, and differential privacy are all methodologies to consider.
Machine learning, while invaluable, relies heavily on data to function effectively. When this data includes personal information, the necessity for stringent data privacy protocols becomes evident. By considering and mitigating the potential disadvantages and challenges in implementing data privacy, organizations can effectively leverage machine learning without compromising the privacy of data subjects.
Artificial Intelligence Master Class
Exponential Opportunities. Existential Risks. Master the AI-Driven Future.
Advantages of Implementing Data Privacy in Machine Learning
The inclusion of data privacy elements in machine learning offers several inherent benefits:
- Enhanced Trust: Potent data privacy measures make machine learning models trusted tools for companies and individuals alike. This trust opens doors to greater data access, thereby refining the model's performance and, subsequently, delivering more accurate insights.
- Risk Mitigation: Adherence to data privacy regulations reduces the risk of non-compliance fines and reputational damage. Companies using machine learning models can face serious backlash for perceived misconduct concerning data privacy.
- Improved Data Quality: Data privacy encourages data evaluation and cleansing, thus reducing inaccuracies in the data input, and results in higher-confidence output.
- Ethical Standards: As social consciousness increases, consumers place more value on ethical practices, including data privacy. Upholding these standards can boost your company's public perception and customer loyalty.
Disadvantages of Implementing Data Privacy in Machine Learning
While crucial, data privacy in machine learning comes with certain challenges:
- Data Quality: Stripping datasets of personally identifiable information might affect the quality of data and the resulting predictions.
- Complex Regulations: Keeping track of intricate and dynamic privacy regulations across varying jurisdictions can be taxing.
- Cost Implications: Implementing data protection, encryption, and compliance tools in machine learning protocols can be expensive.
- Latency: Ensuring privacy can slow down data processing, increasing latency in the provision of machine learning insights.
Take Action
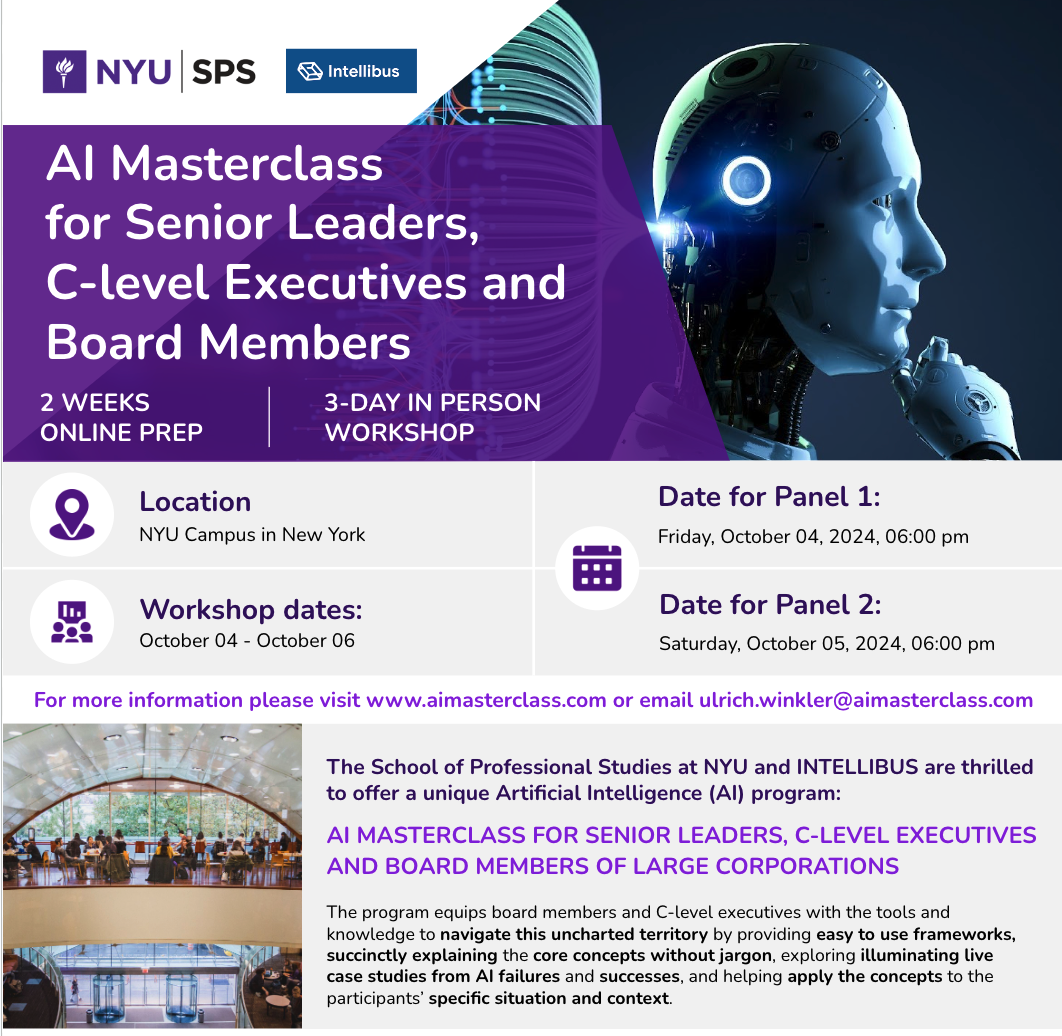
Download Brochure
- Course overview
- Learning journey
- Learning methodology
- Faculty
- Panel members
- Benefits of the program to you and your organization
- Admissions
- Schedule and tuition
- Location and logistics