What is Deep Learning for Natural Language Understanding?
Deep Learning for Natural Language Understanding Explained
In the spectrum of artificial intelligence technologies, Deep Learning for Natural Language Understanding (NLU) is a tool utilized to automate the recognition, understanding, and interpretation of human language in a valuable way. This system leverages complex deep neural networks to extract meaningful information from textual data, paving a way for more sophisticated human-machine interaction.
Key Characteristics of Deep Learning for NLU
Rich Data Processing: Deep learning provides extensive capacity for the processing and interpretation of natural language data, enabling the deciphering of context, sentiment, and intent within a text, and abundant semantic relationships amongst words.
Model Behaviour: Deep learning models can effectively capture implicit language rules, and, unlike traditional models, do not require manual feature extraction which underscores their ability to learn independently.
High Accuracy: Using deep learning for NLU enhances the accuracy of language understanding thanks to its ability to grasp the multitude of layers within language data.
Scalability: The performance of deep learning scales proportionally with the volume and quality of the data, and the computational power of the system.
Continuous Learning: Unlike static systems, deep learning models continually learn, adjusting the understanding of language patterns based on new incoming data.
Deep learning for NLU has found its immense potential being leveraged in several industries, from the automotive sector with speech recognition applications, to customer service with chatbots, and in the health sector for processing and interpreting medical and diagnostic reports.
Artificial Intelligence Master Class
Exponential Opportunities. Existential Risks. Master the AI-Driven Future.
Advantages of Deep Learning for NLU
Enhanced Accuracy: Deep learning models can achieve impressive accuracy rates in language understanding by recognizing conversational speech, dialects, accents, slang, and even emotions. This makes them indispensable tools in diverse domains like customer service, where understanding customer intent can significantly enhance service quality.
Reduced Time: Implementing deep learning models eliminates the need for manual rule-creation and feature extraction, thus reducing the time and resources invested in training the model.
Adaptive: Deep learning for NLU is highly adaptive as it continually learns and adjusts to new language inputs or changes, recognizing updated data inputs and trends, thus staying relevant and effective.
Scalability: Deep learning scales proportionally with the growth in data, providing consistent performance regardless of the size of the language data.
Cost-Effective: Deep learning systems offer a cost-effective choice. By employing these systems, businesses save on resources which would have been used in manual rule creation, or on hiring experts to train these systems.
However, despite several merits, Deep Learning for NLU is not devoid of certain challenges:
Disadvantages of Deep Learning for NLU
Expensive Computation: Deep learning models require vast computational resources, making them a more expensive option, especially for smaller businesses with limited resources.
Lack of Explicit Control: Unlike rule-based models, users of deep learning models do not have explicit control over how the system forms conclusions, which can sometimes lead to unpredicted results.
Data Dependency: The success of these models is directly proportional to the quality and volume of the data available. Without a substantial amount of quality data, these models might not perform at their best.
- Ethical and Security Concerns: With deep learning-powered Natural Language Processing being ubiquitous; issues related to privacy, security, and ethical implications have raised considerable concern.
Implementing Deep Learning for NLU
Successful implementation of Deep Learning for NLU requires careful planning, extensive research, and consideration. Several companies offer pre-trained models that can be customized according to specific business requirements. Moreover, a deep-dive analysis of factors like cost-effectiveness, hardware requirements, data availability, ethical and security concerns, etc. plays a pivotal role in designing an ideal solution for the organization's needs.
By leveraging the power of deep learning for natural language understanding, organizations can realize new possibilities in various domains, from customer service and sentiment analysis to content recommendation, and much more. It's all about harnessing the power embedded in our natural language to drive actionable intelligence, innovation, and growth.
Take Action
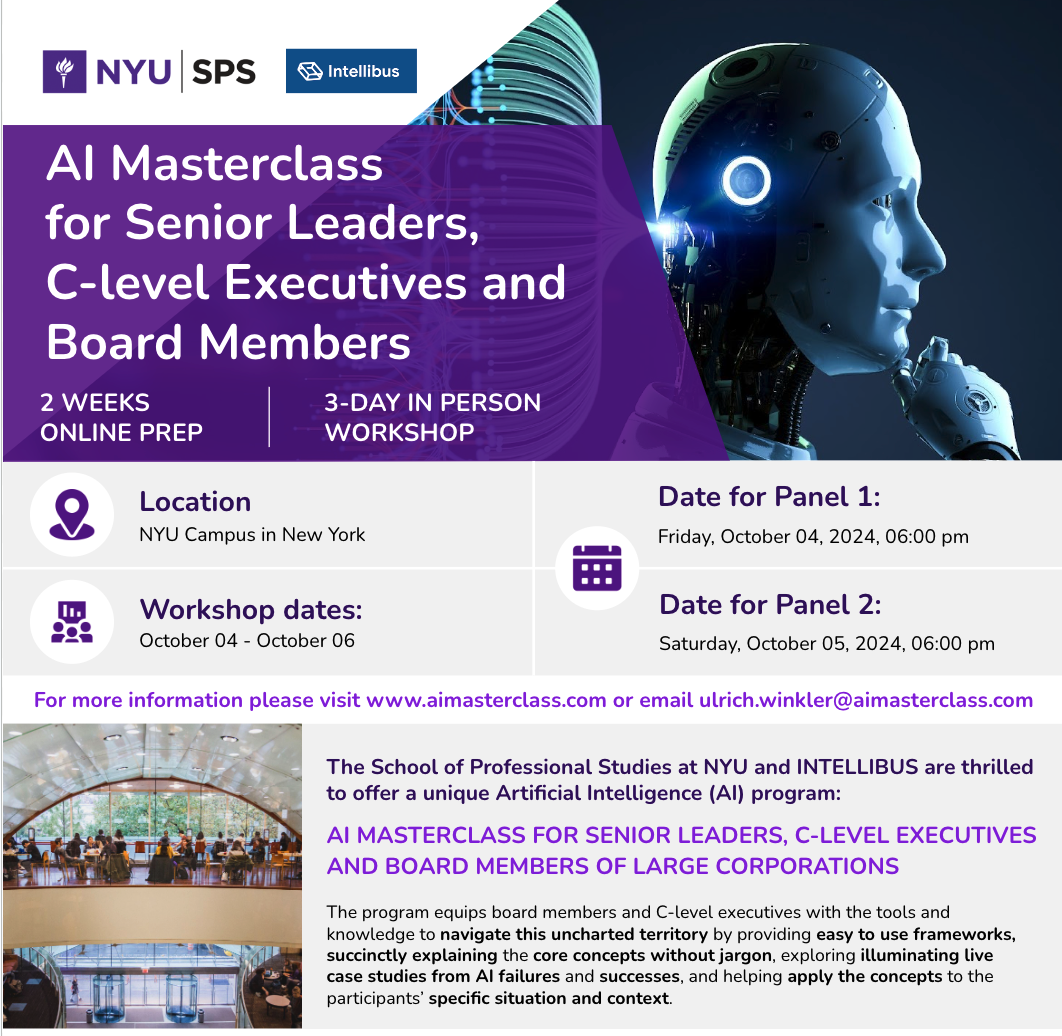
Download Brochure
- Course overview
- Learning journey
- Learning methodology
- Faculty
- Panel members
- Benefits of the program to you and your organization
- Admissions
- Schedule and tuition
- Location and logistics