What is Deep Learning Optimization?
Deep Learning Optimization
Deep learning is a subfield of machine learning that utilizes multiple layers of artificial neural networks to model and comprehend complex patterns and relationships in data. Optimizing deep learning models, therefore, becomes a crucial task for better performance, precise output, and efficient resource utilization. This article explores the concept of deep learning optimization, its importance, and factors affecting it.
What is Deep Learning Optimization?
Deep learning optimization revolves around improving and refining a deep learning model to ensure that it performs at its best. It involves choosing and adjusting various parameters such as learning rate, batch size, number of epochs, optimizer, momentum, among others to improve the learning process, computational efficiency, and overall performance of the model.
Key Characteristics of Deep Learning Optimization
Diverse parameters: Deep learning optimization requires a careful tweaking of a broad range of parameters. This allows the model to learn effectively, provide accurate results and in the short time.
Efficiency: Optimization makes deep learning models computationally efficient. By tuning the model's parameters, we can optimize the training process to consume less time and computational resources.
High Accuracy: Optimized deep learning models generally perform better in terms of accuracy. The tweaking of certain parameters like learning rate and optimizer can have a substantial improvement in the model's predictive output.
- Customization: The optimization of deep learning models often requires a custom approach. Different models may need different types of optimization based on factors such as their complexity, size of data, hardware infrastructure, among others.
Deep learning optimization is a challenging and significant aspect of model development. Careful evaluation, strategic planning, and meticulous experimentation are crucial to successful deep learning optimization. A thorough understanding of the trade-offs involved in each parameter and optimization technique helps to improve a model's performance as per the requirements. Further, optimization creates an efficient deep learning model that provides accurate predictions more quickly, using less computational resources, which is indeed a boon for organizations in their AI journey.
Deep learning optimization involves understanding and tweaking numerous parts of an AI model, from its structure and parameters to its environment and other outside factors. The type of optimization also depends on the problem at hand as well as the specific needs and constraints of the organization or project. Therefore, each optimization process is unique.
Artificial Intelligence Master Class
Exponential Opportunities. Existential Risks. Master the AI-Driven Future.
Advantages of Deep Learning Optimization
Improved performance: By adjusting parameters and implementing optimization techniques, we can significantly improve the model's performance.
Reduced training time: Optimization can help to reduce the time taken to train the models. Techniques such as adjusting the learning rate, batch size, choosing the appropriate optimizer can greatly decrease the training time.
Better resource utilization: Optimizing deep learning models typically results in better utilization of memory and processing power, leading to cost-efficiency.
- Faster deployment: Optimized models usually have a faster prediction time and hence can be deployed more quickly in real-time applications.
Disadvantages of Deep Learning Optimization
Potential overfitting: Over-optimization can lead to overfitting, where the model works exceptionally well on the training data but poorly on new, unseen data.
Requires expertise: Fine-tuning parameters and other optimization techniques require a high level of skill and expertise.
Increases complexity: Incorporating different optimization techniques may increase the complexity and dimensionality of the model.
Ultimately, despite the potential challenges, deep learning optimization represents substantial potential in advancing AI and machine learning applications. By improving the performance and accuracy of our AI models, we can provide more robust, efficient, and reliable solutions for various industries, thereby enhancing our capacity to drive innovation and growth in the AI field.
Take Action
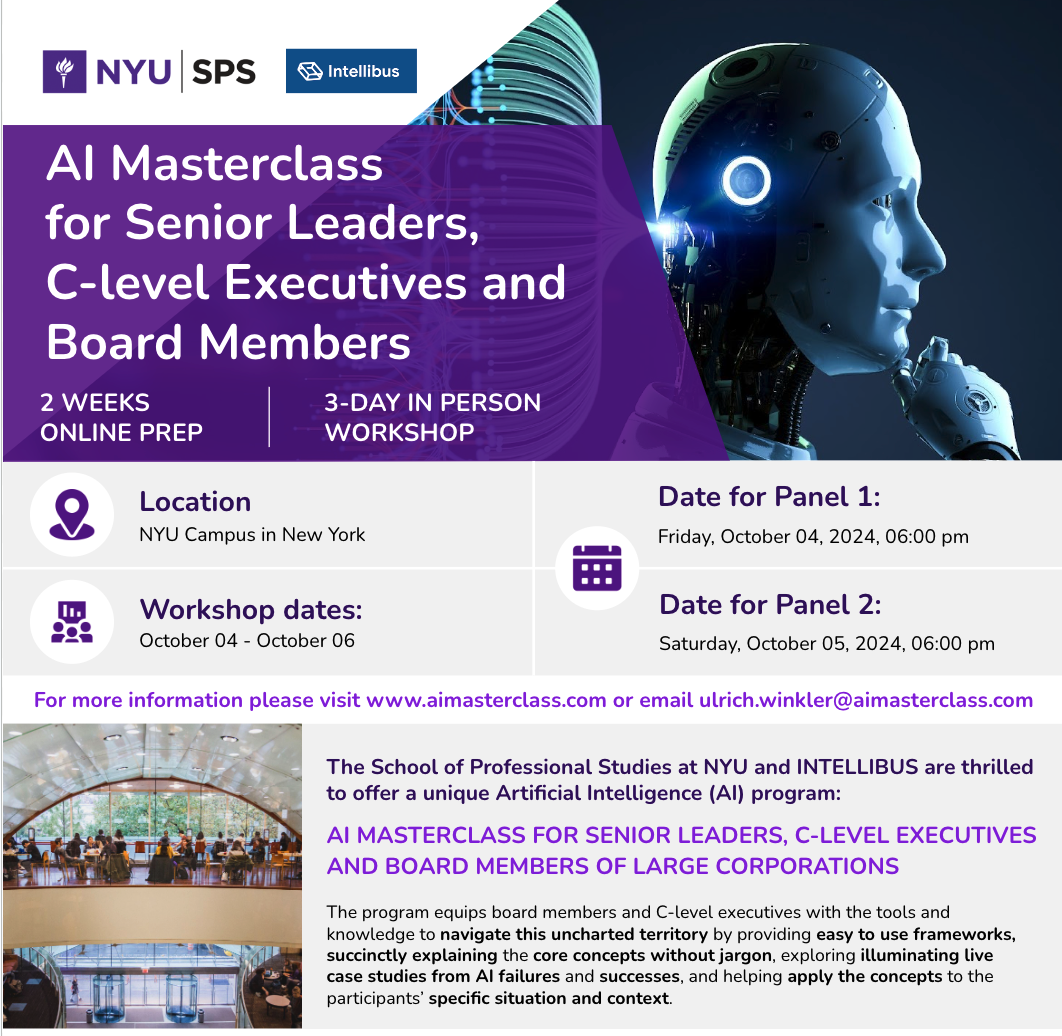
Download Brochure
- Course overview
- Learning journey
- Learning methodology
- Faculty
- Panel members
- Benefits of the program to you and your organization
- Admissions
- Schedule and tuition
- Location and logistics