What is Deep Neural Networks?
Deep Neural Networks Explained
Deep neural networks, also known as DPGNNs, are an advanced and sophisticated type of artificial intelligence (AI) that works by mimicking the functionality of a human brain to solve complex problems. These networks, designed to model high-level abstractions in data, have become increasingly crucial to machine learning and AI.
Primary Features of Deep Neural Networks
Deep neural networks can be characterized by their unique features:
- Hierarchical Layers: Deep neural networks operate using multiple layers, each of which focuses on different aspects and levels of data input and allows for intricate outputs.
- Multitude of Neurons: Each of these layers is composed of numerous interconnected artificial neurons or processing nodes. These nodes work in unison to process information and produce output.
- Adaptive Learning: Each node uses an assigned weight during processing that is adjustable, facilitating the learning process for the machine.
- Large Data Volumes: Deep neural networks are designed to work with vast amounts of data, allowing for deeper and more accurate analysis.
Managing and Implementing Deep Neural Networks
The creation and execution of a deep neural network requires careful planning and an in-depth understanding of its capabilities. The first step involves establishing the problems or tasks that the network is expected to solve and then determining the type of data required. Next, the network's layers and nodes must be appropriately structured and interconnected.
One must also plan for regular assessment and updating of the network to adapt to new challenges and changes in the data landscape. When properly implemented, deep neural networks can offer unparalleled insights and solutions.
While they come with some unique challenges, deep neural networks also offer unmatched capabilities and benefits. With careful planning and understanding, they can be a significant asset to organizations in myriad fields, driving insights, efficiencies, and advancements that would be otherwise unattainable.
Artificial Intelligence Master Class
Exponential Opportunities. Existential Risks. Master the AI-Driven Future.
Benefits of Deep Neural Networks
Deep neural network technology is widely embraced thanks to several inherent advantages that include:
- Sophisticated Computing: Deep neural networks are capable of handling tasks far more complex than traditional computing methods. They can process vast amounts of data, discern patterns, and learn from their inputs and outputs.
- Efficient Problem-Solving: With the model founded on the human brain's neural network, Deep Neural Networks use unsupervised learning and can navigate intricate problems with ease and efficiency.
- Scalability: As the network learns, it gets more efficient at processes, evolving its performance, accuracy, and functionality. It's a technology that can scale and adjust according to the growing needs of the company.
- Critical in Machine Learning and AI: Deep neural networks are at the core of advancements in machine learning and AI. From advanced data analytics to predictive algorithms, these networks are proving crucial in numerous domains.
- Immediate Access to Strategic Insights: Although the system requires a comprehensive initial setup, once functional, deep neural networks can quickly provide deep insights and strategic predictions.
However, alongside the benefits, it's crucial to acknowledge the potential setbacks and challenges this type of AI possesses.
Challenges of Deep Neural Networks
- Understanding Potential Biases: A significant challenge with deep neural networks lies in their inability to recognize biases in the data being processed. This could lead to skewed outcomes and misguided insights.
- Complex Understanding: Despite their capabilities, deep neural networks' inner workings can become challenging to comprehend and explain, making them less accessible to everyone.
- High Resource Requirement: Developing, implementing, and running deep neural networks requires substantial computational power and memory.
- Data Dependency: The efficiency and accuracy of a deep neural network is dependent on the quality and volume of data it has access to. Inaccuracies or insufficiencies in data can affect the overall performance.
Take Action
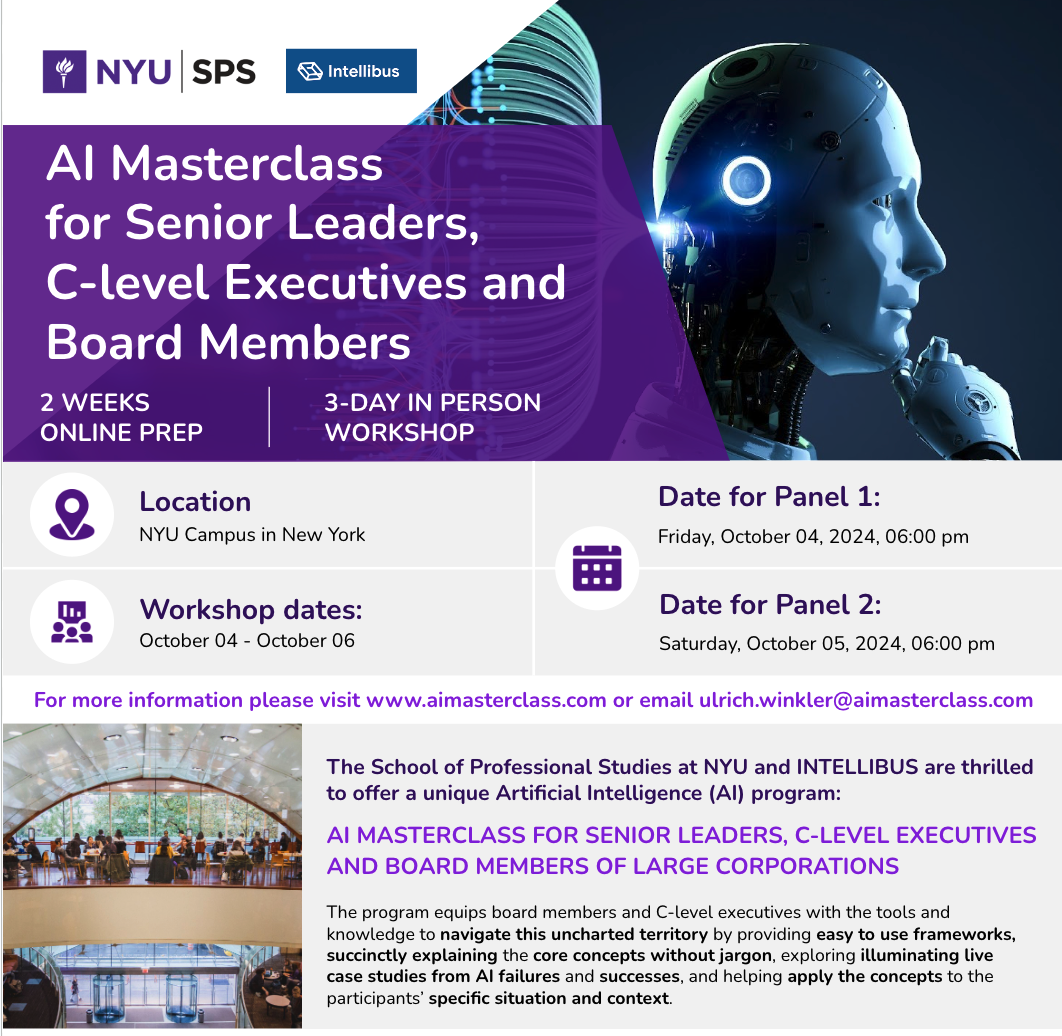
Download Brochure
- Course overview
- Learning journey
- Learning methodology
- Faculty
- Panel members
- Benefits of the program to you and your organization
- Admissions
- Schedule and tuition
- Location and logistics