What is Distributed AI Systems?
What are Distributed AI Systems?
Distributed Artificial Intelligence (DAI) systems are a class of models that entail a collaborative network of artificial intelligence agents designed to solve complex problems. Unlike a stand-alone AI model, a DAI system operates across various nodes/networks to execute tasks simultaneously thereby optimizing performance and elevating efficiency. This unique design caters to an array of applications, spanning from data analysis to decision-making, all the while accommodating the intricate requirements of various users or organizations.
Distributed AI Systems Exhibit Several Key Characteristics:
- Wide Scalability: DAI systems can expand or contract easily according to the needs of the task at hand or the computational resources available.
- Flexible Design: The architecture of DAI systems can adapt to varied tasks and problems, thanks to their constituent elements - the AI agents - which can individually learn, adapt, and perform.
- Minimal Centralization: DAI systems operate without a single central node, thus epitomizing a distributed approach to problem-solving.
- Automated Decision-Making: DAI systems consist of autonomous agents that simulate human-like decision-making processes, often functioning in unison to solve a complex problem.
- Resource Optimization: Owing to their distributed nature, DAI systems can utilize resources more efficiently than centralized systems. Consequently, this makes DAI systems highly resilient and cost-effective.
Industries worldwide are fast recognizing the potential of Distributed AI systems for their adeptness at providing high computational power, improved reliability, and enhanced scalability.
Implementing Distributed AI Systems
Implementing DAI systems requires a well-considered plan that starts with a detailed assessment of the organization's needs and the selection of suitable AI agents. Subsequently, cost-benefit analysis and vendor evaluation ensure alignment with company requirements. Moreover, efficient coordination strategies, robust security measures, and consistency controls are integral for a successful implementation. Bear in mind, the deployment of DAI systems should be closely monitored and refined as needed.
Artificial Intelligence Master Class
Exponential Opportunities. Existential Risks. Master the AI-Driven Future.
Advantages of Distributed AI Systems
Distributed AI systems boast several inherent advantages, including:
- Cost-Effectiveness: Given that DAI systems spread the computational load across multiple nodes, they are generally more affordable than centralized systems. Besides, they provide the added benefit of optimizing hardware usage and reducing the need for infrastructure expansion.
- High Durability: The distributed nature of these systems boosts their resilience and results in higher system durability due to the absence of a single-point failure.
- Improved Performance: The simultaneous operation of multiple independent AI agents provides DAI systems with superior performance as it accelerates problem-solving and optimizes system throughput.
- Increased Adaptability: Unlike traditional AI models, DAI systems can adapt easily to changing requirements or environments, mainly due to their flexible architecture.
- Scalability: DAI systems can efficiently cope with an increase in workload by merely adding more nodes to the system. This feature makes them perfect for businesses expecting long-term growth.
- Enhanced Data Management: They provide a more comprehensive data analysis due to its capability of handling vast amounts of distributed data.
Disadvantages of Distributed AI Systems
Despite their many benefits, DAI systems also have some limitations:
- Complexity: The system's distributed nature can lead to increased complexity in managing and maintaining the system.
- Costs of Coordination: Operating numerous AI agents together demands resourceful coordination strategies. It could potentially drive up costs and affect the overall performance of the system.
- Security Challenges: The distributed architecture could potentially increase the surface for cyber attacks, and this necessitates more robust security measures.
- Data Consistency: Maintaining consistency across all nodes can be a challenge, primarily when they operate concurrently.
- Limited Control: With autonomous agents, users may not have complete control over the system’s behavior, making the system less predictable.
In conclusion, Distributed AI systems, despite some challenges, offer considerable potential for organizations dealing with complex tasks and big data. In such a context, their proper implementation can deliver substantial benefits including high scalability, superior performance, and enhanced resilience.
Take Action
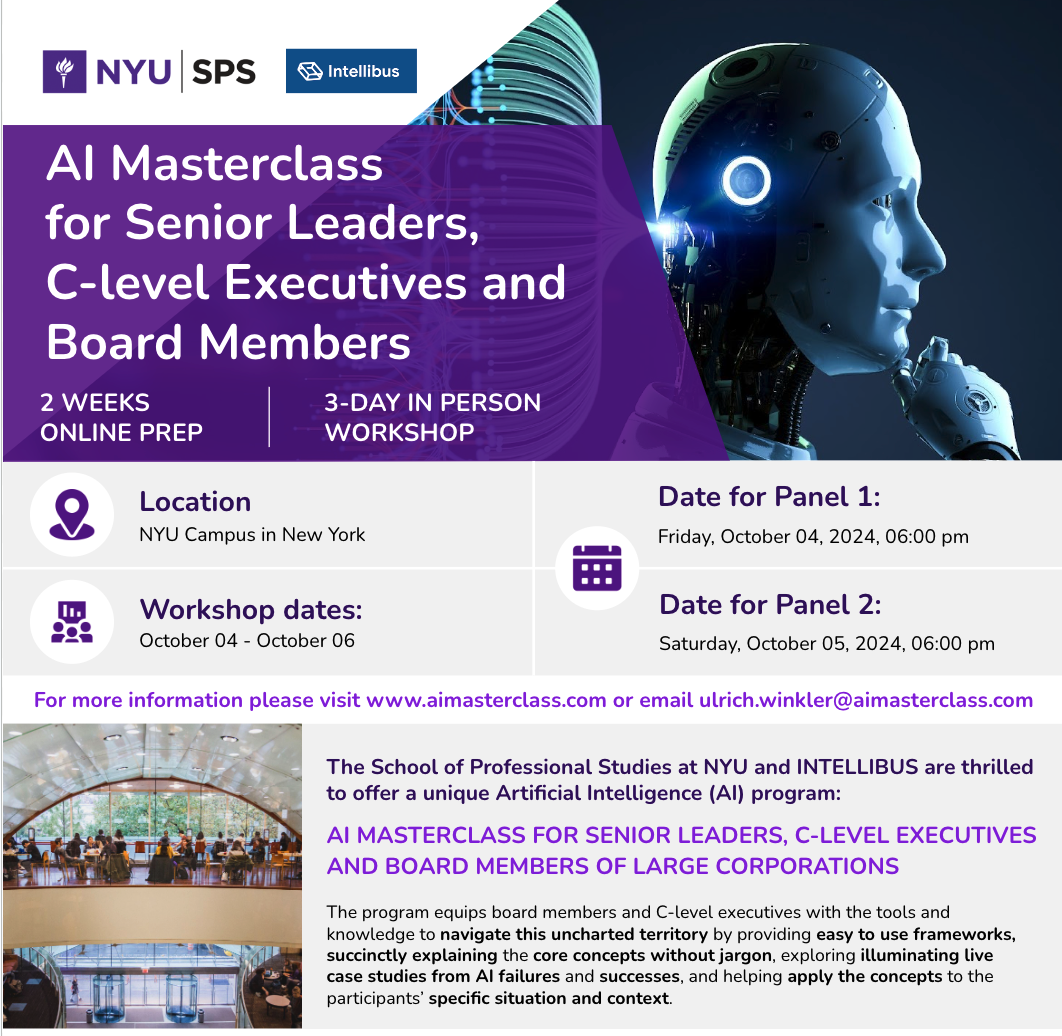
Download Brochure
- Course overview
- Learning journey
- Learning methodology
- Faculty
- Panel members
- Benefits of the program to you and your organization
- Admissions
- Schedule and tuition
- Location and logistics