What is Ensemble Methods for Anomaly Detection?
What is Ensemble Methods for Anomaly Detection?
Ensemble Methods encompass a range of algorithms designed for anomaly detection, that leverage the power of multiple models to enhance the overall system's predictive performance. Generally, ensemble methods use multiple detectors, each detecting anomalies in its own unique manner. The final result is determined by aggregating the results of these individual detectors.
Ensemble Methods for Anomaly Detection exhibit a series of key characteristics:
- Multitude of Detectors: Ensemble methods utilize multiple models or detectors to identify anomalies. This approach greatly enhances the accuracy and precision of detection.
- Aggregated Results: The outcomes of various models are aggregated to give a final result. The method for aggregation could involve majority voting, calculated averages or more complex algorithms.
- Robust Performance: Leveraging multiple models makes ensemble methods robust when faced with complex, high-dimensional data sets.
- Incremental Learning: Ensemble methods can evolve and adapt over time with the introduction of new data. This enables the model to continuously learn and improve its accuracy.
- Diversity: Diversity among ensemble members can lead to significant error reduction as it allows capturing a broader view of potential anomalies.
Different industries often employ Ensemble Methods for Anomaly Detection for its robustness in handling complex data, higher detection precision, and ability to learn and adapt over time.
Artificial Intelligence Master Class
Exponential Opportunities. Existential Risks. Master the AI-Driven Future.
Advantages of Ensemble Methods for Anomaly Detection
Several inherent advantages make ensemble methods a go-to solution for many organizations. These include:
- Increased Precision: The collective decision-making process enhances the chances of identifying true anomalies, thereby improving precision and minimizing false positives.
- Robust Performance: By leveraging multiple models, ensemble methods handle intricate, high-dimension datasets effectively, providing robust performance even in a complicated environment.
- Adaptability: With incremental learning, ensemble methods can adapt to new data, learn from it, and improve detection accuracy over time. This capacity to evolve with more information is a significant advantage.
- Scalability: Ensemble methods can handle a broad range of anomalies. Their scalable nature makes them a robust tool for growing organizations.
- Error Reduction: By deploying various models with different perspectives, ensemble methods can reduce error rates compared to the utilization of a single model.
Disadvantages of Ensemble Methods for Anomaly Detection
Despite numerous advantages, certain drawbacks of ensemble methods merit consideration:
- Computational Complexity: Employing multiple models can increase computational complexity. As a result, the processing time may escalate, and ensuring proper handling of resources might become a challenge.
- Overfitting: There is a potential risk of overfitting the model if the individual detectors are too similar.
- Lack of Interpretability: Due to the combination of several models, ensemble methods can be black boxes, making it difficult to understand their decision-making process.
- Hidden Costs: The increased complexity might result in hidden costs associated with resources, model management, and maintenance.
Implementation of Ensemble Methods for Anomaly Detection
Implementing ensemble methods involves a thorough understanding of the organization's needs and meticulous selection of suitable individual detectors. Subsequently, the manner of aggregating their results should be decided based on the specific scenario. In addition, the implementation calls for careful management and evaluation of the models to ensure the robust handling of resources and optimal system performance.
Successful application of ensemble methods for anomaly detection requires constant monitoring post-deployment, addressing any emerging issues, and fine-tuning the models to ensure maximal effectiveness and efficiency. When accurately implemented and managed, ensemble methods can provide a robust solution to anomaly detection, enabling businesses to take swift, preventive actions and make more informed decisions based on data.
Take Action
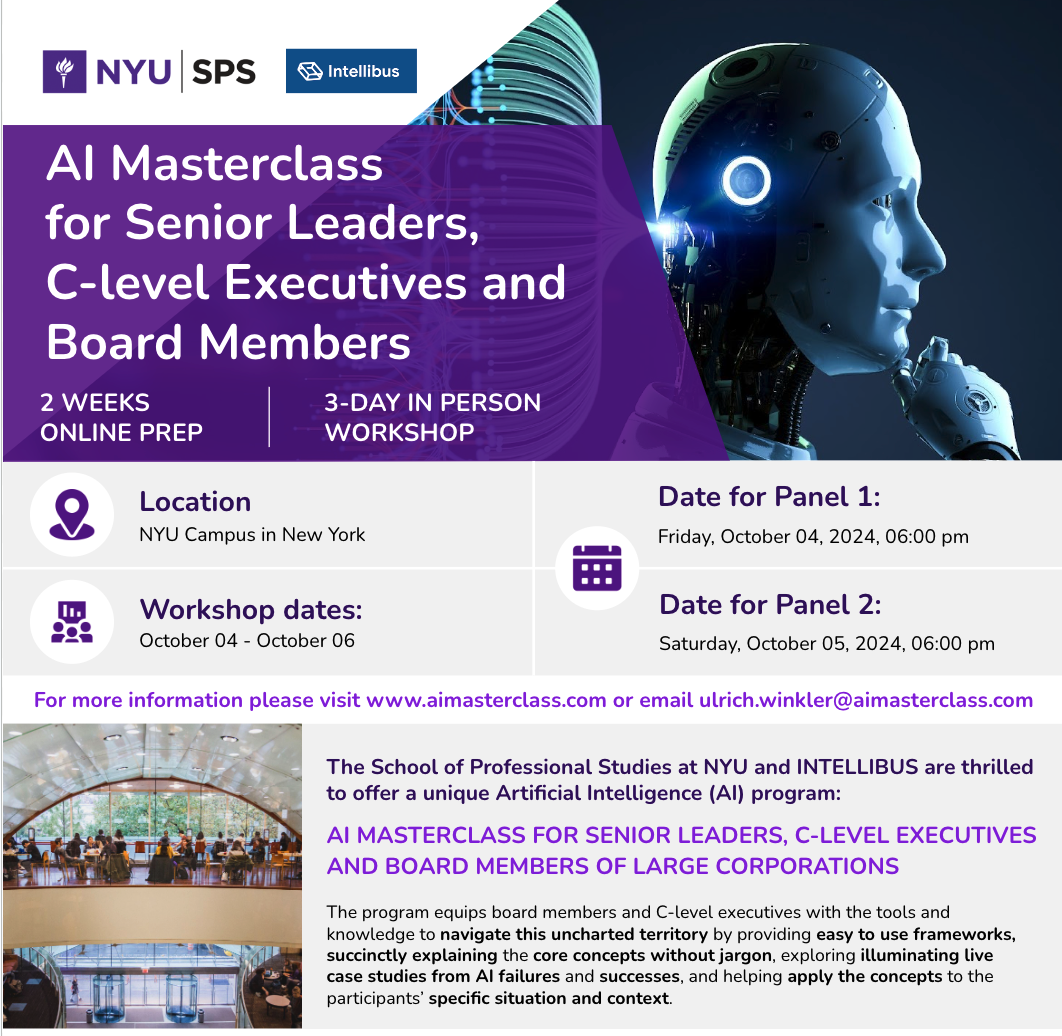
Download Brochure
- Course overview
- Learning journey
- Learning methodology
- Faculty
- Panel members
- Benefits of the program to you and your organization
- Admissions
- Schedule and tuition
- Location and logistics