What is Explainable AI?
What is Explainable AI?
Explainable AI, also known as XAI, is the AI sector concerned with making machine learning models and their results understandable and interpretable to humans. As Artificial Intelligence (AI) becomes more sophisticated and we increasingly use it to make high-stakes decisions, the need for AI transparency is heightened. As a methodology, Explainable AI aims to fulfill this need, facilitating transparency and trust in machine learning applications.
Key Attributes of Explainable AI
- Transparency: As its core feature, Explainable AI provides insight into the working mechanism of AI applications, allowing users to understand and interpret the decision-making process.
- Interpretability: XAI models are designed to produce interpretable results, allowing users to understand and confirm the logic behind the outcomes.
- Traceability: Users can trace the decision-making path of an AI model, illuminating how the model arrived at a particular conclusion.
- Trust-building: As it demystifies AI's internal workings, Explainable AI promotes user trust, fostering user acceptance and widespread utilization of AI.
- Compliance Support: XAI aids in ensuring compliance with regulations requiring transparency and avoiding bias in automated decision-making solutions; this includes legislation like GDPR.
Industry-wide, adoption of Explainable AI is increasing, fueled by its potential to foster a better understanding of the mechanisms underlying AI solutions, encourage trust in these technologies, and support regulatory compliance.
Explainable AI Implementation
Implementing Explainable AI involves several processes, including requirements analysis, model selection, resource allocation, and regulatory compliance assessment. The process also demands meticulous planning and direction to ensure a successful implementation.
The adoption and implementation of Explainable AI is vital. It promotes trust, compliance, and optimization in the use of AI technologies. However, organizations should also be aware of the challenges associated with its implementation and devise strategies to mitigate potential issues. The goal is to strike a balance between achieving AI performance and transparency. Making AI outcomes understandable to users is a critical step towards the democratization and widespread acceptance of AI technologies.
Artificial Intelligence Master Class
Exponential Opportunities. Existential Risks. Master the AI-Driven Future.
Advantages and Drawbacks of Explainable AI
- Boosting Trust: Explainable AI enables users to comprehend why a decision was made by a system, fostering trust in AI technologies. Trust becomes particularly critical in high-stakes applications, such as healthcare, where AI decisions significantly impact individual lives.
- Collaboration Improvement: Understanding how AI reaches its conclusions assists AI developers and users to collaborate more effectively, by illuminating areas for model refinement and result verification.
- Regulatory Compliance: Explainable AI aids in meeting regulatory requirements for transparency and non-discrimination in automated decision-making processes.
- Quality Control: As it unveils the decision-making rationale, Explainable AI supports error identification and model optimization.
- Democratization of AI: By making AI interpretable, XAI fosters user acceptance, thus promoting AI's widespread use and democratization.
Despite its numerous advantages, the use of Explainable AI presents several challenges:
- Complexity: Intricate AI models often possess an inverse relationship between performance and interpretability.
- Resource-intensive: Implementing Explainable AI demands significant resources, which may divert attention from other critical project aspects.
- Limitations in Explanation Scope: Although XAI aims for full transparency, some model attributes may remain inexplicably complex.
- Implications for IP and Competition: Full model transparency might jeopardize the intellectual property of organizations developing AI solutions.
Take Action
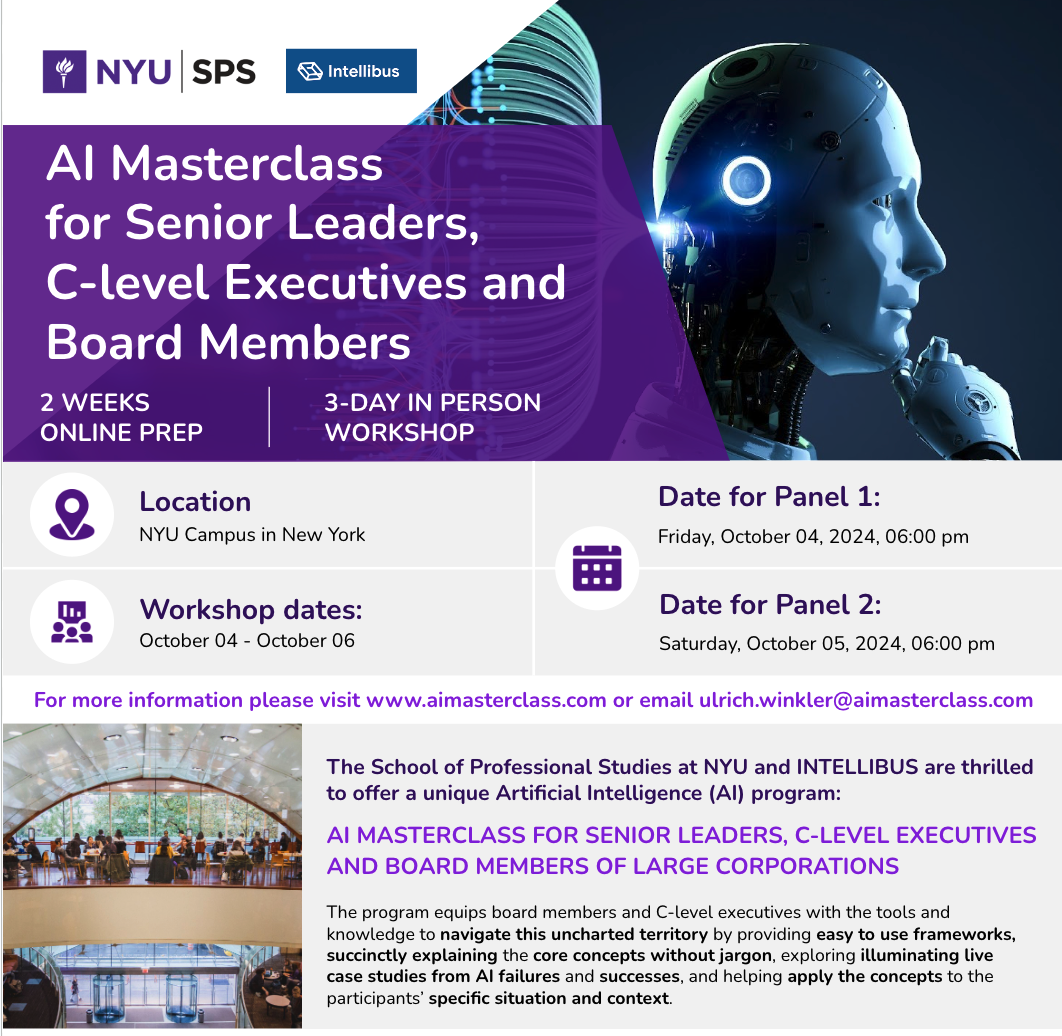
Download Brochure
- Course overview
- Learning journey
- Learning methodology
- Faculty
- Panel members
- Benefits of the program to you and your organization
- Admissions
- Schedule and tuition
- Location and logistics