What is Image Enhancement with Deep Learning?
The Impact of Deep Learning on Image Enhancement: Revolutionizing Image Quality
Image enhancement is a crucial process in image processing, playing a central role in effective visualization and analysis. In the modern era, employing deep learning techniques for image enhancement has emerged as a superior solution given their sophisticated application of artificial intelligence. Deep learning-based image enhancement technologies enjoy applicability across diverse sectors, including health care, meteorology, defense, and retail among others.
Characteristics of Image Enhancement Using Deep Learning
- Superior Image Quality: Deep learning drives enhancements of image quality, enabling automatic adjustment of brightness, color, and contrast
- High Efficiency: Processing time of images for enhancement using deep learning algorithms is noticeably reduced, making it highly effective
- Adaptability: Deep learning models adapt to various lighting conditions and backgrounds, enhancing image quality across various scenarios
- Automatic Learning: Deep learning techniques continually evolve and learn from new data, improving performance over time
- Ability to Handle Complex Scenarios: Deep learning-based models can handle complicated scenarios, including noise reduction, super-resolution, and photorealistic imaging rendering
Artificial Intelligence Master Class
Exponential Opportunities. Existential Risks. Master the AI-Driven Future.
Advantages and Drawbacks of Image Enhancement Using Deep Learning
- Improved Accuracy: Deep learning algorithms significantly improve the accuracy of image enhancement, offering superior results compared to traditional techniques
- Automated Process: Deep learning enables automation of image enhancement process which not only saves time but also allows for handling large volumes of data
- Real-time Image Enhancement: Deep learning algorithms can improve image quality in real time, significantly expanding potential use cases
- Enhanced Decision Making: Improved image quality facilitates better analysis, thereby enhancing decision-making processes
Despite the myriad of advantages, there are certain limitations associated with image enhancement using deep learning:
- Resource Requirement: Deep learning algorithms for image enhancement require substantial computational resources and power, leading to increased costs
- Dependency on Quality of Training Data: The quality and accuracy of image enhancement relies heavily on the quality of training data. If the training data is biased or inadequate, it may negatively impact the results
- Complexity and Understanding: Deep learning algorithms are complex, which might necessitate specific skill sets and knowledge base to understand and operate efficiently
- Unreliable in Changing Environments: Rapid changes in environmental conditions or unique imaging conditions might affect the performance of deep learning models in image enhancement
Taking steps to mitigate these challenges is critical in leveraging the benefits of deep learning for image enhancement. This can be achieved through capacity planning, provision of adequate computational capabilities, high-quality training datasets, and thorough understanding of the algorithms at play.
Step by step implementation of Image Enhancement with Deep Learning
Implementing deep learning for image enhancement demands careful planning and execution:
- Assessment: One should first evaluate the specific image enhancement requirements and performance expectations
- Procurement of High-Quality Data: Sourcing appropriate, high-quality images to train the deep learning model is paramount
- Model Selection: Based on the identified requirements, a suitable deep learning model should be selected and configured accordingly
- Training: Following model selection, one must then train the deep learning algorithm using the collected dataset
- Evaluation and Testing: After training, it's essential to evaluate the model's performance against a set of test data to verify model’s reliability and efficacy
- Fine-Tuning and Deployment: After satisfactory performance validation, final tuning of the algorithm is performed for optimal performance before deployment
Deep learning-based image enhancement is transforming the landscape of image processing with improved accuracy, efficiency, and automation. By understanding the advantages, addressing potential limitations, and implementing a structured, well-planned approach, organizations can harness the power of deep learning to significantly enhance image quality.
Take Action
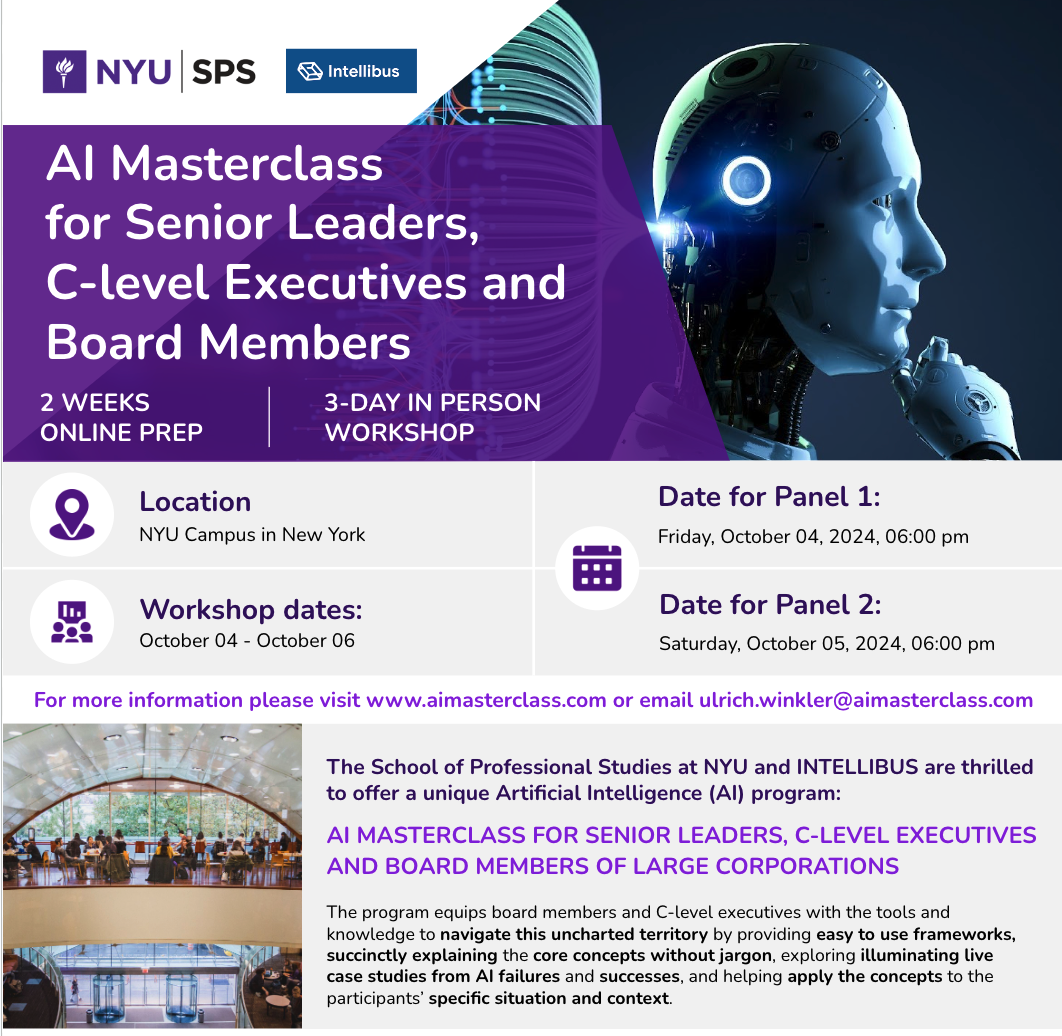
Download Brochure
- Course overview
- Learning journey
- Learning methodology
- Faculty
- Panel members
- Benefits of the program to you and your organization
- Admissions
- Schedule and tuition
- Location and logistics