What is Joint Entity Recognition and Linking?
Understanding Joint Entity Recognition and Linking
Joint Entity Recognition and Linking (JERL), an essential facet of Natural Language Processing (NLP), serves as an effective tool for the identification of entities or named objects within a given text. Furthermore, it effectively links them to corresponding entries in a knowledge graph or a database of structured information. The key feature of JERL is that it performs Entity Recognition (ER) and Entity Linking (EL) tasks simultaneously rather than separately, leading to increased accuracy and context understanding.
Notable Characteristics of Joint Entity Recognition and Linking
JERL exhibits several key traits that make it invaluable to various sectors:
- Contextual Accuracy: JERL uses the context of the entire text to identify and categorize entities, leading to superior accuracy.
- Simultaneity: ER and EL tasks integrate, allowing JERL to accomplish both simultaneously. This integrated approach leads to enhanced precision compared to sequentially performing these tasks.
- Extensibility: The vast knowledge bases that JERL links to are usually extensible, facilitating further contextual exploration.
- Increased Efficiency: JERL reduces complexities and inaccuracies associated with the traditional separate execution of ER and EL tasks.
- Deep Understanding: By recognizing and linking entities, JERL yields a deeper understanding of the text narrative.
Strategies for Successful JERL Implementation
A successful implementation of JERL requires careful planning and a series of robust strategies. Initially, one needs to choose the right models for incorporation in a unified architecture. Following model selection, the team should focus on gathering a diverse and high-quality data set for training. Evaluation of the database also constitutes a crucial task to ensure accurate data.
Further, it's critical to analyze computational requirements and plan resources accordingly to prevent unforeseen expenditures. Moreover, it's equally important to conduct regular audits to identify weaknesses in the system and arrange subsequent revisions, as needed. Post-deployment reviews and continued refinements also comprise the crux of a successful implementation.
Joint Entity Recognition and Linking, with their distinct advantages, necessitate a well-planned approach for implementation. By understanding the complexities and potential challenges upfront, organizations can successfully integrate JERL to their computational linguistic endeavors, yielding enhanced efficiency, accuracy, and a deeper understanding of text narratives.
Artificial Intelligence Master Class
Exponential Opportunities. Existential Risks. Master the AI-Driven Future.
Advantages and Drawbacks of Joint Entity Recognition and Linking
Organizations and researchers within NLP and related fields benefit from numerous inherent advantages of JERL, including:
- Efficiency: JERL simultaneously performs ER and EL actions through a unified architecture, eliminating the need for separate algorithms and redundant processes.
- Improved Accuracy: Through the simultaneous execution, JERL enhances the accuracy of the ER and EL tasks, thereby reducing incorrect entity identification and linking.
- Contextual Understanding: Comprehensive view of the text is obtained due to the simultaneous recognition and linking of entities, increasing the understanding of the context and narrative.
- Comprehensive Database: JERL avails a vast array of structured data from extensible knowledge bases, increasing the depth of information available for analytics.
- Reduced Manual Intervention: Given its automation, JERL minimizes manual annotation needed, thus saving time and reducing errors.
Despite these advantages, there are challenges associated with the use of JERL:
- Complexities of Integration: Integrating ER and EL into a unified model can be complex, requiring significant technical expertise.
- Dependency on Databases: The functionality depends on the quality and extent of the database or knowledge graph used. If the database lacks details or exhibits errors, it directly affects the effectiveness of JERL.
- Insufficient Training Data: Due to the need for diverse and high-quality training data, the lack thereof may hamper the model’s performance.
- Computational Requirements: Given the simultaneous execution of tasks, JERL models may require higher computational power, which can influence cost-effectiveness.
Take Action
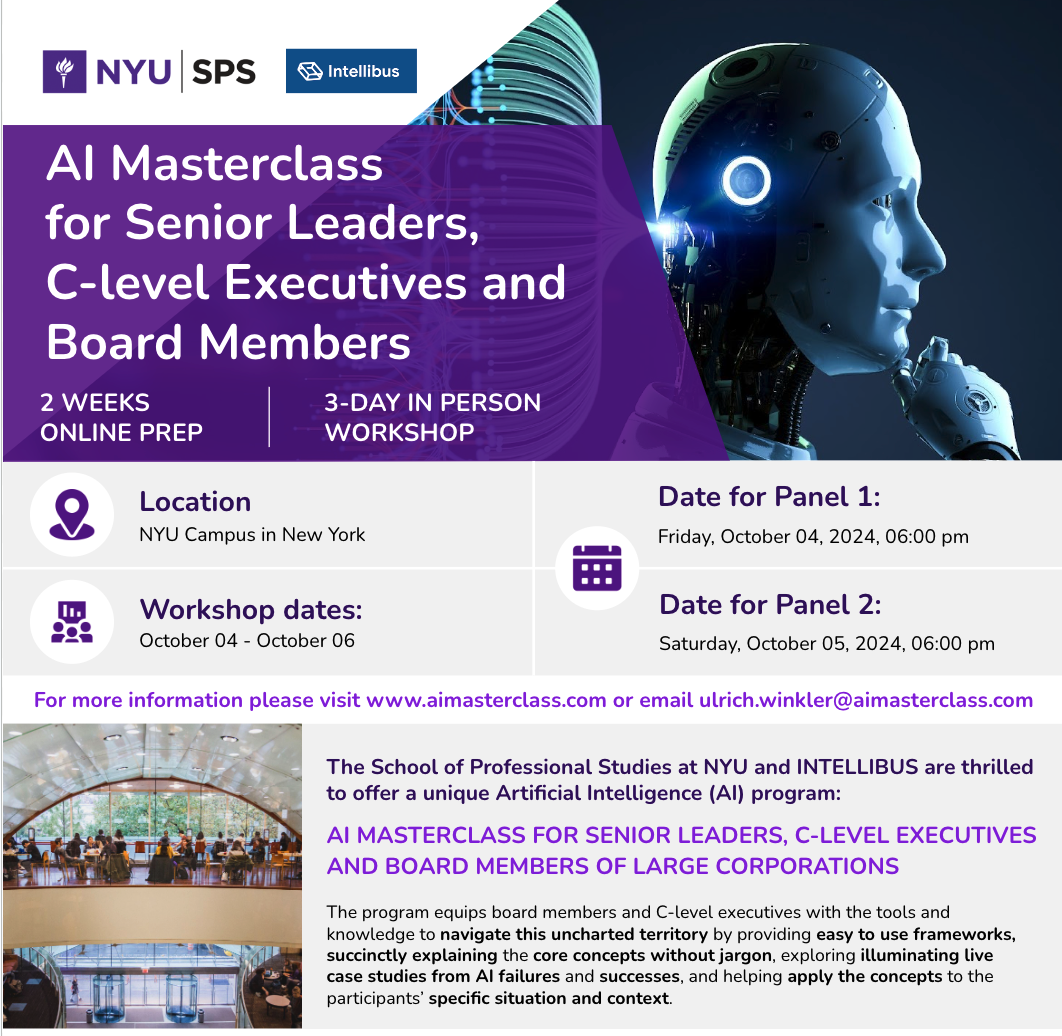
Download Brochure
- Course overview
- Learning journey
- Learning methodology
- Faculty
- Panel members
- Benefits of the program to you and your organization
- Admissions
- Schedule and tuition
- Location and logistics