What is Knowledge Extraction Techniques?
Understanding Knowledge Extraction Techniques
Knowledge extraction techniques, commonly referred to as KE techniques, entail a systematic approach utilized to derive essential information from data. These techniques target structured, unstructured, or even semi-structured data, aiming to reveal meaningful insights for decision-making and other key processes within an organization.
Key Characteristics of Knowledge Extraction Techniques:
- Wide Applicability: KE techniques find usage in numerous applications across diverse domains, including the health sectors, financial industries, marketing, and customer management, among others.
- Actionable Insight Generation: These techniques generate critical, actionable insights from vast, complex data, thereby allowing businesses and organizations to leverage these insights to drive growth.
- Advanced Algorithms: Knowledge extraction techniques often deploy advanced algorithms, including machine learning, to locate patterns, correlations, and other relevant features within data sets.
- Real-time Data Analysis: Most KE techniques provide the capacity for real-time data analysis, facilitating immediate insight generation.
- Scalability: KE algorithms are designed to handle big data, entailing an ability to scale with the progression of data growth.
Implementation of Knowledge Extraction Techniques
Implementing KE techniques necessitates a well-thought-out approach and process. An initial undertaking would involve a comprehensive evaluation of data quality and extent to ensure its usefulness and relevance. This should then be followed by a careful selection of the KE technique most appropriate for the given data and organizational objectives. These steps are significant in ensuring optimized outcomes from the KE process.
At the core, the implementation of knowledge extraction techniques pivots on various elements including the quality of data, efficiency of algorithms, and ability to generate actionable insights. The process demands consistency and close-monitoring to verify the accuracy of extracted knowledge and evaluate its impact on an organization’s decision-making processes.
When effectively employed, Knowledge Extraction techniques possess the prowess to enable businesses to explore complex data landscape and extract meaningful insights. Through these, organizations can fathom the concealed patterns within their data, subsequently driving more insightful decisions and achieving operational excellence.
Artificial Intelligence Master Class
Exponential Opportunities. Existential Risks. Master the AI-Driven Future.
Advantages of Knowledge Extraction Techniques
Knowing and adopting Knowledge Extraction techniques offers several potential benefits:
- Improved Decision-Making: KE techniques turn raw data into valuable insights, which are key in making informed strategic decisions. This capability to decipher complex data into understandable and applicable information allows organizations to lessen the uncertainties associated with decision-making processes.
- Cost-Effective: Employing KE techniques could prove more cost-effective than other data analysis techniques. They reduce the need for manual data analysis, thus saving time and effort.
- Efficiency: Complex algorithms underpinning KE techniques often provide efficient data analysis, converting big data into meaningful information quickly.
- Unveiling Hidden Patterns: These techniques allow businesses to identify hidden patterns and trends within data, affording a richer understanding of various business facets.
- Risk Management: The insights derived from KE techniques can support risk management by predicting potential risks and issues.
Disadvantages of Knowledge Extraction Techniques
While knowledge extraction techniques provide significant value, they are not without drawbacks:
- Data Quality Dependent: The validity and quality of the extracted knowledge heavily depend on the quality of the data used. Inaccurate or incomplete data can, therefore, lead to misleading results.
- Complexity: The algorithms used in KE techniques can sometimes be complex, requiring a certain level of skill and expertise to operate effectively.
- Privacy Issues: KE techniques often involve both personal and sensitive data analysis, which could potentially compromise privacy if not appropriately managed.
- Time-Consuming: Although KE techniques are generally efficient, the initial stages that include data pre-processing and cleaning can be time-consuming.
- Lack of Explainability: There is a level of transparency and interpretability concerns, especially when using black-box type machine learning algorithms that provide limited insights into how they derive the final results.
Take Action
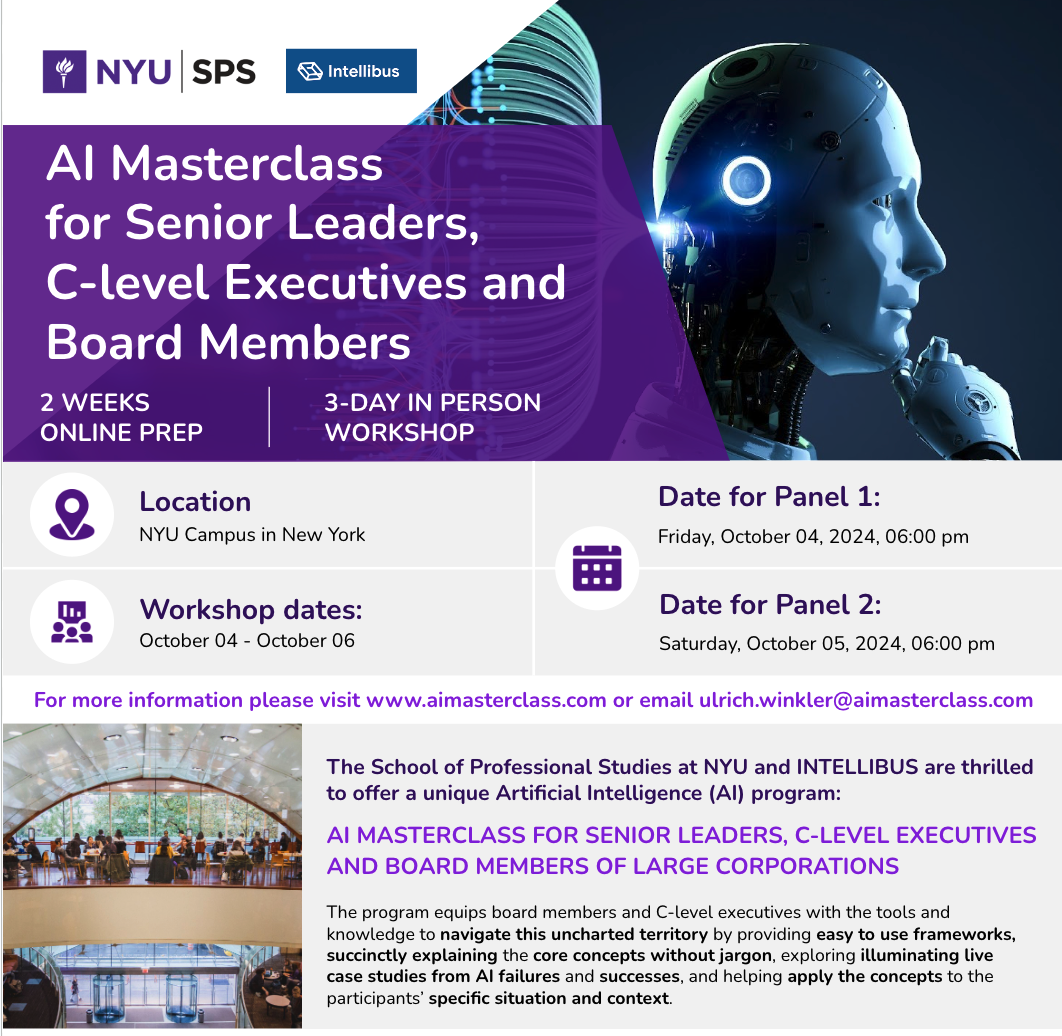
Download Brochure
- Course overview
- Learning journey
- Learning methodology
- Faculty
- Panel members
- Benefits of the program to you and your organization
- Admissions
- Schedule and tuition
- Location and logistics