What is Knowledge Graphs for Semantic Search?
What are Knowledge Graphs for Semantic Search?
In the realm of search technology, Knowledge Graphs for Semantic Search, often abbreviated as KGSS, serve as one of the most advanced tools, refining search results to provide more relevant and meaningful information. These are vast plots of interconnected data points, specifically designed to comprehend real-world entities and their relationships. Unlike traditional keyword-based searches, which return results based on word presence and frequency, knowledge graphs for semantic search focus on concept understanding and context awareness.
KGSS showcases essential features:
- Broad Accessibility: Knowledge graphs are typically open-source and accessible to users to extract and analyze data from different domains.
- Comprehensive Data: KGSS comprises a diverse range of data types including structured, semi-structured, and unstructured data, ensuring a comprehensive understanding of information.
- Extensive Connectivity: Knowledge graphs carry a massive network of interlinked data offering a holistic view of information which goes beyond linear connections.
- Automated Learning: KGSS supports machine learning techniques which enable predictive analytics, data inference, and automatic data completion.
- Improved Data Quality: Their ability to link a wide range of diverse data steers towards improved data quality and reduced inconsistency.
- Cost-Beneficiary: Leveraging existing knowledge graphs often proves more cost-efficient than designing customized data models from scratch.
Industries widely deploy Knowledge Graphs for Semantic Search to improve search efficiency, provide a personalized user experience, enhance decision-making protocols, help optimize advertising strategies, and assist in semantic SEO.
Implementation of KGSS
Integrating knowledge graphs for semantic search requires a well-planned approach. This involves deep analysis
of organizational needs, careful selection of the appropriate knowledge graph and semantic search tools, calibration of the tools to ensure alignment with the organization's data models, and regular monitoring to identify and rectify any discrepancies, inconsistencies or gaps in data. Given the technical complexity, organizations may also need to invest in training for employees or hire experts specializing in KGSS. With appropriate implementation, KGSS can significantly enhance the organization's search efficiency and decision-making capabilities.
Artificial Intelligence Master Class
Exponential Opportunities. Existential Risks. Master the AI-Driven Future.
Advantages of KGSS
- Cost-Effective: Being open-source, KGSS are usually free of cost, hence, significantly reducing the expenses associated with buying or developing custom models.
- Enhanced Search Relevance: Semantic search focuses on understanding the searcher's intent and the contextual meaning of terms used in the search, leading to more refined and relevant search results.
- Predictive Analysis: The interconnected data structure of KGSS, coupled with machine learning techniques, supports predictive modeling. This allows for forecasting trends and outcomes based on historical data.
- Personalized User Experience: The semantic understanding proffers a personalized user experience by catering to individual's specific needs and preferences.
- Automated Data Completion: Knowledge graphs have the ability to self-learn and auto-complete missing data points, hence improving data quality and consistency.
- Ongoing support: Being open-source, KGSS usually have vast support communities that provide regular upgrades, patches, troubleshooting resources, user guidelines, and best practices.
Disadvantages of KGSS
- Limited Customizability: As knowledge graphs are often open-source, the scope of customizing them according to specific organizational requirements is usually limited.
- Technical Complexity: Understanding and implementing KGSS requires significant technical expertise that could prove complex and overwhelming to non-tech users.
- Data Privacy Concerns: As KGSS involves connecting and integrating diverse data assets, data privacy concerns may arise.
- Dependence on Community Support: With open-source KGSS, the responsibility of updates, patches, and troubleshooting falls mainly on the community, which could lead to delays or non-availability of assistance.
- Integration Challenges: Metadata standards vary across different fields, which can present significant challenges when integrating diverse datasets.
Take Action
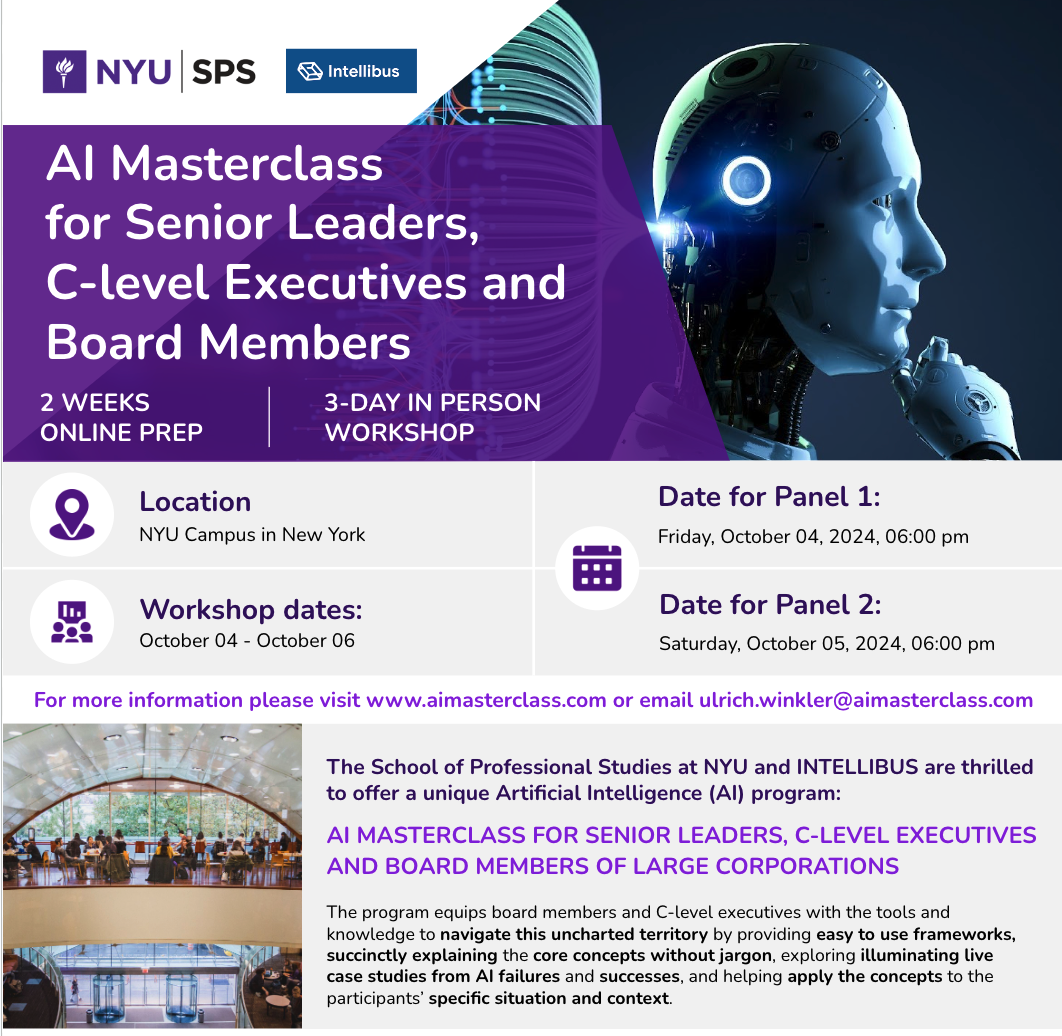
Download Brochure
- Course overview
- Learning journey
- Learning methodology
- Faculty
- Panel members
- Benefits of the program to you and your organization
- Admissions
- Schedule and tuition
- Location and logistics