What is Latent Semantic Analysis for Text?
Understanding Latent Semantic Analysis for Text
Latent Semantic Analysis (LSA) is a groundbreaking computational technique utilized to extract and analyze contextual meaning from text data. It unearths the inherent semantic structure within text by discerning patterns in the relationships amidst words and clauses in unstructured data.
Characteristics of Latent Semantic Analysis (LSA)
Unsupervised Learning: LSA operates without prior human intervention, automatically identifying and recognizing patterns within raw text data, distinguishing its unsupervised learning ability.
Dimension Reduction: The technique usefully condenses high-dimension data (words within a text) into a lower-dimensional space, called the semantic space. The purpose lies in reducing computation complexity while retaining key semantic connections.
Dissimilarity Detection: Through its unique statistical distribution approach, LSA can find dissimilarities among words and group like-terms and concepts together.
Semantics Extraction: LSA uncovers underlying semantic aspects that are not obvious at first glance. It analyzes contexts and patterns of word usage, determining associated meanings that humans might overlook.
Scalability: Regardless of the language or the data volume, LSA algorithm can process significant amounts of raw text restating its scalability feature.
Latent Semantic Analysis is leveraged in industries like research, marketing, and customer service for a wide range of applications, like search engine optimization, customer feedback analysis, content examination, and more.
Success with Latent Semantic Analysis
The key to successful LSA implementation involves a careful understanding of the technique, clear identification of data objectives, and a meticulous selection of analysis tools. Adequate attention to post-analysis interpretation is also crucial for leveraging LSA's insights effectively. Lastly, continuous monitoring of the LSA process helps in recognizing potential errors and refining the analysis process over time.
In conclusion, Latent Semantic Analysis is an extraordinarily potent technique that enables computers to understand human language in depth. It uncovers hidden connections and insights that provide a deeper understanding of raw text data. While acknowledging its limitations, the value and enhancement it brings to data analysis are deep and extensive, making it a worthy tool to explore and implement to drive business decisions and strategies.
Artificial Intelligence Master Class
Exponential Opportunities. Existential Risks. Master the AI-Driven Future.
Benefits of Latent Semantic Analysis
Latent Semantic Analysis accrues several significant advantages:
Enhanced Content Understanding: LSA imparts computers the capability to understand and process human language, deciphering more profound themes hidden in the bulk of text data.
Efficient Data Processing: Able to handle huge volumes of data and condense intricate data structures, LSA is efficient and quick in processing raw data into useful insights.
Contextual Analysis: LSA can detect and understand the context in which words are used, valuably improving the accuracy and depth of text analysis.
Advanced Pattern Detection: By reducing the dimensionality of data, deeper recognition of complex patterns becomes possible.
Robustness: LSA can manage synonyms and polysemy without explicitly requiring disambiguation rules, which makes it a robust tool for content analysis.
Pitfalls of Latent Semantic Analysis
While proposing substantial benefits, a few downsides of Latent Semantic Analysis are:
Limited Interpretability: The conceptual understanding LSA generates might not always line up with human comprehension, posing a challenge for manual verification and adjustment.
Handling of Negations: Difficulty arises when LSA deals with negated structures due to the lexical level at which the system operates.
Need for Large Corpus: A voluminous corpus is required to perform a comprehensive encoding of the meanings which might be a limitation in cases with less data.
Difficulty with Homonyms: Words with multiple meanings can create difficulties as LSA does not always discern between different contextual interpretations.
Independence of Word Order: The inability to take the order of words into account can induce inaccurate analysis.
Take Action
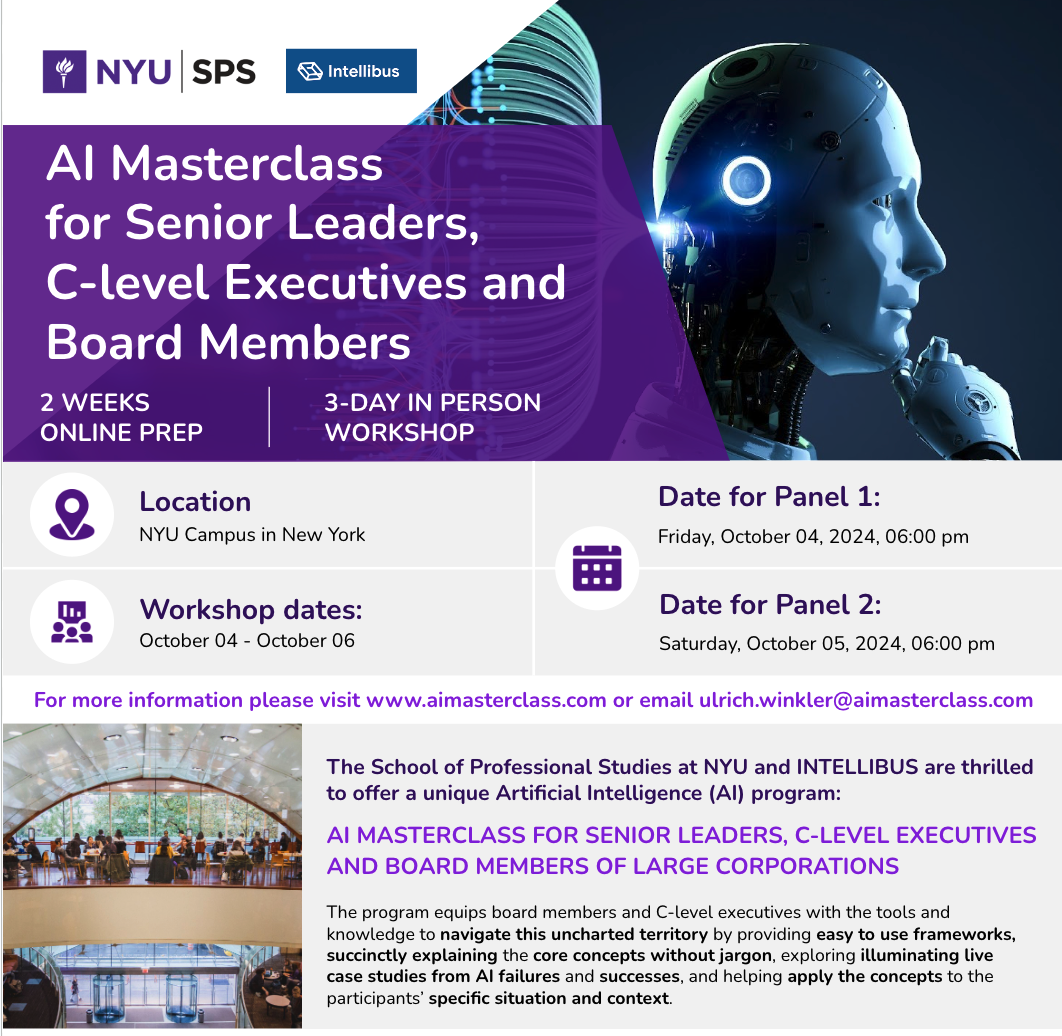
Download Brochure
- Course overview
- Learning journey
- Learning methodology
- Faculty
- Panel members
- Benefits of the program to you and your organization
- Admissions
- Schedule and tuition
- Location and logistics