What is Linear Regression Models?
Linear Regression Models: A Comprehensive Insight
Linear regression is a statistical methodology involving a dependent variable and one or more independent variables that find usage in predicting or estimating real-world phenomena. This approach is widely used in applications involving predictive analysis, applied sciences, social sciences, among many others.
Characteristics of Linear Regression Models
Standard Form: Linear regression models are represented in a standard equation form - Y = a + bX + e, where Y is the dependent variable, X represents the independent variable(s), 'a' is the intercept, 'b' is the slope, and 'e' is the error term.
Intercept and Slope: The intercept (a) represents the value of Y when X is equal to zero, while the slope (b) indicates the change in Y for each unit change in X.
Error Term: The error term (e) in the model captures the difference between the actual and predicted values of Y, accommodating for the unexplained variation in the model.
Fitted Values and Residuals: Fitted values are predicted values of the dependent variable, while residuals represent the difference between the observed and predicted values.
Implementing Linear Regression Models
The application of linear regression models demands cautious execution. It starts with defining the research question, identifying the dependent and independent variables, acquiring and cleaning data, followed by model development, interpretation, diagnostics, and validation. Typically, sensitivity analyses are undertaken to ensure robustness of the results. Finally, model insights are used to inform decision-making.
Linear regression models are a powerful tool in the hands of analysts and researchers who understand their strengths and limitations. They enable us to discover patterns, predict future outcomes, and uncover relationships in our data. However, prudent usage demands a understanding of their assumptions, careful interpretation of their output and acknowledgement of their inherent limitations.
Artificial Intelligence Master Class
Exponential Opportunities. Existential Risks. Master the AI-Driven Future.
Strengths of Linear Regression Models
The utilization of linear regression models comes with an array of strengths:
- Interpretability: The parameters in a linear regression model are straight-forward to interpret and understand. The model allows one to quantify and isolate the impact of each predictors on the outcome variable.
- Efficiency: Linear regression models are quick to develop and require less computational resources compared to other complex predictive models.
- Flexibility: These models can accommodate a large number of predictors and can be adapted to handle non-linear relationships using transformations.
- Transparency: The assumptions underlying linear regression add transparency, making it easier to diagnose and fix issues with the model.
Limitations of Linear Regression Models
Despite its strengths, one should not overlook the limitations:
- Assumptions: Linear regression models rest on several assumptions such as linearity, independency, normality and equal variance of the residuals. Violation of these assumptions can lead to erroneous interpretations.
- Outliers and Multicollinearity: Linear regression models are sensitive to outliers and multicollinearity, which can distort the estimates.
- Overfitting: The flexibility to include many predictors can sometimes lead to overfitting, where the model performs well on the training data, but fails with new data.
- Influence of Independent Variables: The linear regression model assumes that every independent variable is uncorrelated, which might not always be the case in real-world scenarios.
Take Action
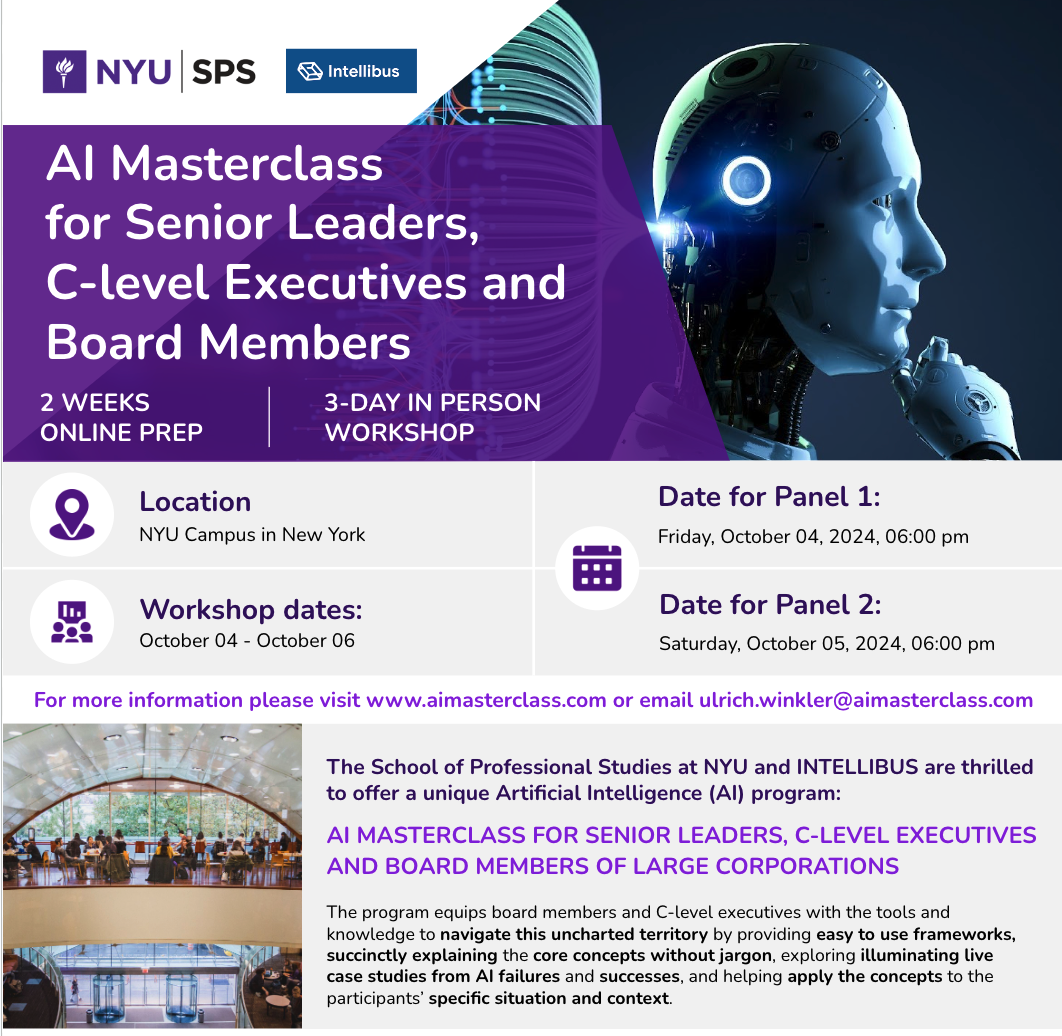
Download Brochure
- Course overview
- Learning journey
- Learning methodology
- Faculty
- Panel members
- Benefits of the program to you and your organization
- Admissions
- Schedule and tuition
- Location and logistics