What is Machine Learning for Predictive Maintenance?
Machine Learning for Predictive Maintenance: An Overview
Machine Learning (ML) for predictive maintenance represents innovative software solutions that leverage mathematical algorithms to forecast equipment downtimes in various industries. These applications rely primarily on historical data to estimate future outcomes – the very crux of predictive maintenance. Unlike custom software development tailored to particular user or organization requirements, Machine Learning for predictive maintenance addresses broader needs across industries.
Key Features of Machine Learning for Predictive Maintenance
Machine Learning solutions for predictive maintenance boast specific traits:
- Broad Reach: Accessible directly from the provider or through various channels, these solutions are widespread and readily available to consumers globally.
- Elaborate Functionality: These applications encompass a rich suite of functionalities, which are meticulously designed and tested to cater to extensive industry needs.
- Minimal Customization: While some degree of customization is viable, the use of ML for predictive maintenance typically involves minimal modifications.
- Provider Support: Companies providing the software solutions also bear the responsibility of maintaining and updating the application and offering technical support, thus ensuring seamless operations.
- Cost Efficiency: Generally, the use of Machine Learning technology for predictive maintenance is more cost-friendly compared to developing a custom solution from the ground up.
Artificial Intelligence Master Class
Exponential Opportunities. Existential Risks. Master the AI-Driven Future.
Benefits of Using Machine Learning for Predictive Maintenance
The use of ML for predictive maintenance comes with an array of advantages:
- Cost-Effectiveness: Owing to its broad user base, the development cost of such machine learning technology is reasonably divided, rendering it economically viable and cost-efficient in comparison to custom-made solutions. This results in a reduction of troubleshooting expenses hence making it financially beneficial.
- Reduced Development Time: As these solutions come ready for deployment, organizations can significantly save on time compared to custom software which requires development from scratch.
- Proven Dependability: ML solutions for predictive maintenance are used across multiple industries which subjects them to a wide range of testing conditions thus ensuring optimal reliability.
- Consistent Updates & Support: Service providers for Machine Learning software for predictive maintenance usually offer continuous support including regular updates, addressing security vulnerabilities and keeping the software up-to-date with technological advancements.
- Integration Ease: The design of most ML applications for predictive maintenance takes compatibility into account, making it easier to integrate with existing systems.
Drawbacks of Using Machine Learning for Predictive Maintenance
While the benefits are numerous, the use of ML for predictive maintenance also presents certain limitations:
- Limited Customization: The software may not meet the unique demands of every user or business, given its design for a broad user base.
- Provider Dependence: Users may become overly reliant on the provider for updates and support, which can pose challenges if the product becomes discontinued or if the company closes down.
- Integration Challenges: Integration with existing systems, especially older infrastructures, might pose challenges despite the software's design aims for compatibility.
- Redundant Features: Some features may be irrelevant to certain businesses, leading to unnecessary complexity and confusion.
- Hidden Costs: Beyond the initial investment, other costs related to licensing, customization, integration, and maintenance may arise.
Implementing Machine Learning for Predictive Maintenance
Effective deployment of ML solutions for predictive maintenance requires a well-planned approach; such as a thorough analysis of the organization’s needs, and a detailed cost-benefit evaluation. This is followed by careful vendor selection to ensure alignment between their product offerings, support services, and company requirements.
As organizations are increasingly maturing in their journey to ML adoption for predictive maintenance, a well-strategized implementation can go a long way toward the successful execution and realization of practical benefits. Additionally, robust provider support ensures that even challenges such as those linked to integration, security and compatibility are addressed promptly. With its capacity to transform maintenance from being merely reactive to becoming proactive, Machine Learning for predictive maintenance represents a powerful tool for organizations across a wide array of industries.
Take Action
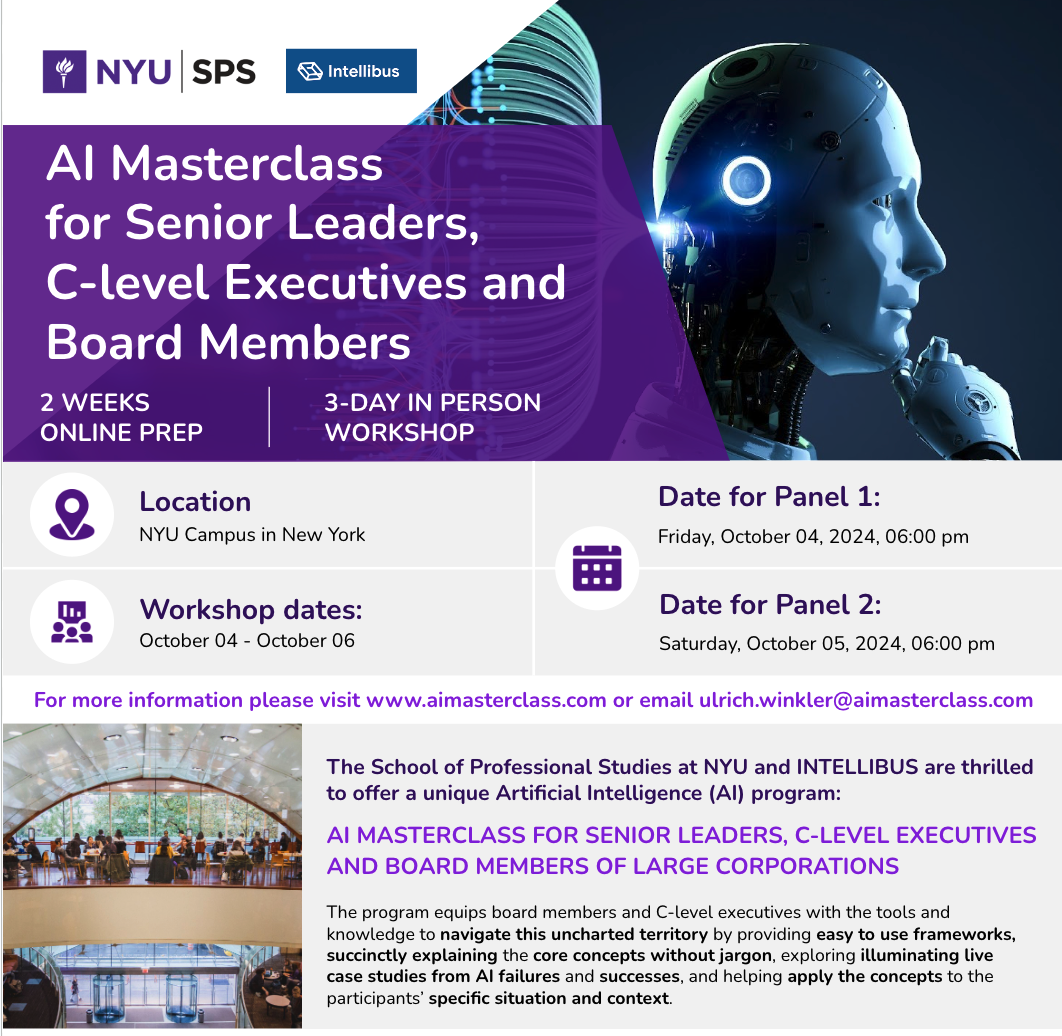
Download Brochure
- Course overview
- Learning journey
- Learning methodology
- Faculty
- Panel members
- Benefits of the program to you and your organization
- Admissions
- Schedule and tuition
- Location and logistics