What is Machine Learning Models?
Understanding Pre-Trained Machine Learning Models
Pre-Trained Machine Learning models, similar to the large-scale applicability of Commercial off-the-shelf (COTS) software, offer ready-to-use and tested data-driven solutions that can be instantly deployed for a wide variety of applications. Instead of creating a machine learning model from scratch, which could be both time-consuming and costly, these algorithms are already trained on a vast amount of data and are ready to provide immediate results.
Characteristics of Pre-Trained Machine Learning Models:
Ease of Use: Machine Learning is a highly specialized field requiring significant expertise and understanding of complex mathematical algorithms. Pre-Trained models are easy to deploy, as the model has already been trained, and is ready to perform its function, reducing the need for extensive technical knowledge.
Standard Features: Pre-Trained Models come with multiple functionalities. They are created to act upon particular data points effectively, reducing the need to tailor custom features for individual tasks.
Low Customization: With Pre-Trained Machine Learning Models, one can expect minimal customization. They are designed to cater to common use-cases rather than particular organizational needs.
Provider Support: The model provider takes care of updates, improvements, and bug fixes, ensuring smooth functioning.
Cost-Effective: Due to their wide audience and applicability, Pre-Trained Models tend to be more cost-effective than building a Machine Learning Model from scratch, particularly for common applications.
Implementation of Pre-Trained Machine Learning Models
Implementing a pre-trained model requires a thorough understanding of the organization's needs and careful selection of the most fitting model. Conducting an in-depth cost-benefit analysis and assessing the model provider's support services is crucial. Monitoring the deployment closely and being open to adjustments according to the organization's specific needs are key elements for successful implementation. As in every technological decision, the advantages and disadvantages must be carefully weighted to ensure a truly beneficial investment.
Artificial Intelligence Master Class
Exponential Opportunities. Existential Risks. Master the AI-Driven Future.
Advantages of Pre-Trained Machine Learning Models
Several benefits make Pre-Trained Models an attractive option:
Cost-Effective: Pre-Trained Models provide a more affordable solution than building a custom model, as development costs are distributed across a wide user base. It results in a cost-effective solution, particularly for businesses lacking the resources and expertise to develop custom Machine Learning Models.
Reduced Development Time: Given such models are ready for deployment, the time taken to develop a model from scratch is eliminated. This immediate availability is beneficial for businesses that require quick data insights.
Reliability: Extensively tested, Pre-Trained Models are exceptionally robust and reliable. They have undergone thorough validation and evaluation across diverse use-cases, ensuring their capabilities under varying conditions.
Regular Updates and Support: Vendors typically provide regular bug fixes and updates for pre-trained models. This support is crucial to patch security vulnerabilities and keep the model updated with the latest technologies and features.
Ease of Integration: Most Pre-Trained Models are designed to be compatible with other systems, making integration quick and easy.
Immediate Access to Advanced Features: Pre-Trained Models give users direct access to advanced features and functionalities, eliminating the costly and time-consuming process of development.
Disadvantages of Pre-Trained Machine Learning Models
Despite the numerous advantages, there are a few limitations:
Limited Customization: Pre-Trained Models may not cater to unique needs or workflows of specific organizations. Lack of customization could limit the application of such models in specialized environments.
Vendor Dependence: Having to rely on the vendor for updates and improvements may create a dependency, particularly if the vendor discontinues the model or ceases operations.
Limited Influence: Users typically have little to no influence over the product roadmap of pre-trained models, and future updates may not align with their specific needs.
- Security and Compatibility: Pre-Trained Models might come with security threats due to their significant usage. Also, any changes made by the vendor might not be compatible with the user's system, resulting in potential compatibility issues.
Take Action
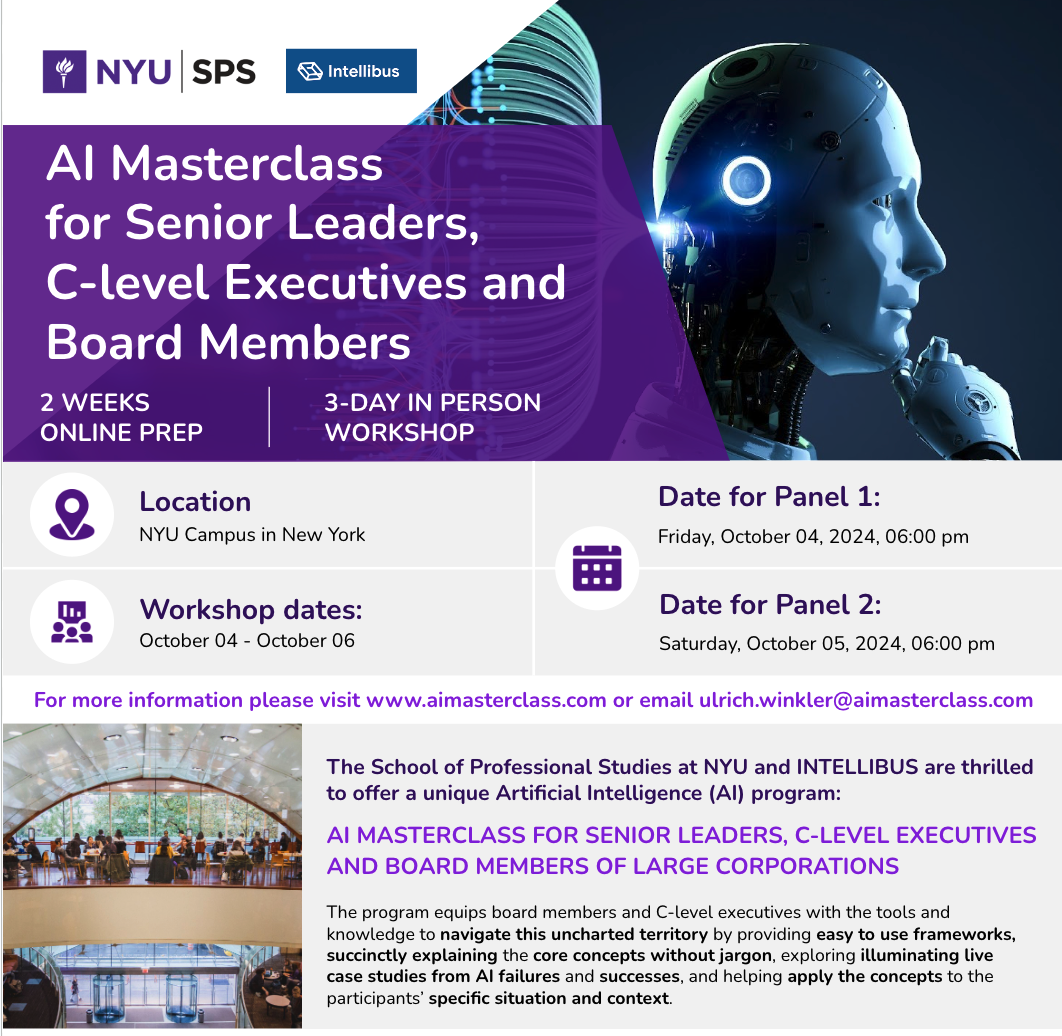
Download Brochure
- Course overview
- Learning journey
- Learning methodology
- Faculty
- Panel members
- Benefits of the program to you and your organization
- Admissions
- Schedule and tuition
- Location and logistics