Back to glossary
What is Meta-Learning for Model Adaptation?
Understanding Meta-Learning for Model Adaptation
Key Characteristics of Meta-Learning:
Artificial Intelligence Master Class
Exponential Opportunities. Existential Risks. Master the AI-Driven Future.
APPLY NOW
Advantages of Meta-Learning for Model Adaptation
- Efficiency: Meta-learning models enable rapid adaptation to new tasks, environments and unknown territories, facilitating adaptability and quick responses in sectors where data is continuously evolving.
- Scalability: In terms of scalability, meta-learning models improve with more tasks, handling the increasing complexity and facilitating growth.
- Performance: Meta-learning can improve performance over time and across tasks, thereby providing robust and reliable model results.
- Cost-Effective: As these models can effectively apply knowledge from prior tasks, the training time for new tasks is significantly reduced, saving resources and costs.
- Flexibility: Meta-learning models offer flexibility, accommodating a variety of tasks and applications across different domains.
Disadvantages of Meta-Learning for Model Adaptation
- Data Dependency: Most meta-learning methods operate under the assumption that tasks are drawn from a shared distribution. If tasks significantly deviate from this assumption, the performance may be undermined.
- Complexity: The complexity of meta-learning models, specifically in terms of computational resources and the time required to train them, can present significant challenges.
- Limited influence: Given its data-driven nature, users of meta-learning models exert minimal to no influence on the model learning process, potentially resulting in less control over outcomes.
Implementing Meta-Learning Models for Model Adaptation
In conclusion, meta-learning for model adaptation serves as a significant leap towards developing AI systems that can learn and adapt from experience, much like humans. By successfully mitigating the limitations, meta-learning can pave the way for more intelligent and adaptable systems.
Take Action
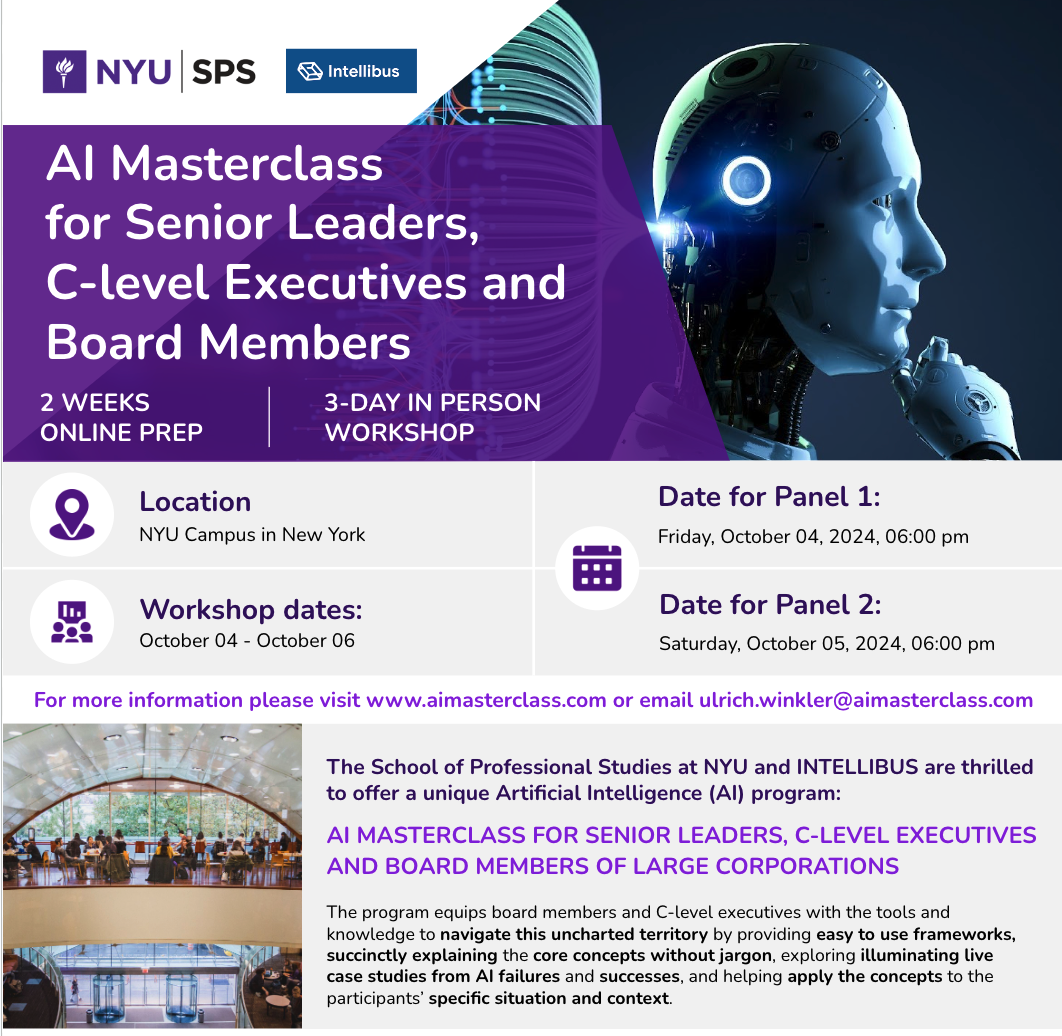
Download Brochure
What’s in this brochure:
- Course overview
- Learning journey
- Learning methodology
- Faculty
- Panel members
- Benefits of the program to you and your organization
- Admissions
- Schedule and tuition
- Location and logistics
Contact Us
I have a specific question.
Attend an Info Session
I would like to hear more about the program and ask questions during a live Zoom session
Sign me up!
Yes! I am excited to join.