What is Multi-Objective Optimization in AI?
What is Multi-Objective Optimization in AI?
Multi-Objective Optimization (MOO) in artificial intelligence, frequently referred to as Multi-objective Problem Solving (MOPs), addresses problems with multiple competing objectives. These are problems where two or more goals exist and advances in one goal may lead to setbacks in another; implying there's usually no single solution that maximizes all the objectives at once.
MOO in AI has critical features:
- Advanced Problem-solving: The spectrum of multi-objective problems covers complex decision-making instances wherein conflict exists between objectives, necessitating trade-offs.
- Smart Decision Making: This technique permits decision-makers to make an informed and intelligent choice from among multiple conflicting options.
- Competing Learning Goals: In the machine learning environment, MOO leverages to harmonize competing learning objectives.
- Diversity of Solutions: It affords the possibility for diverse, yet satisfactory solutions, also known as Pareto optimal solutions.
MOO finds broad usage within various domains due to its capacity for comprehensive problem handling, adaptability in offering multiple solutions, and ability to address real-world complexities.
Implementing Multi-objective Optimization in AI
Successfully implementing MOO requires a thoughtful approach that takes into consideration the complexity of the problem, computational resources, the availability of expert knowledge and the desired outcomes. There are several MOO methods ranging from traditional mathematical programming techniques to modern evolutionary algorithms, each with its unique strengths and limitations. It is crucial to critically evaluate these methods and choose one that best fits the problem at hand.
A structured implementation process typically begins with the identification of objectives and then moves on to method selection, problem-solving, and the interpretation of results. The use of MOO in AI has accelerated progress in addressing real-world problems due to its readiness to handle complexities and offer a variety of solutions. However, seekers of this technique must be prepared to navigate its challenges and requirements for successful application.
Artificial Intelligence Master Class
Exponential Opportunities. Existential Risks. Master the AI-Driven Future.
Advantages of Multi-objective Optimization in AI
Organizations and academicians look positively towards the MOO approach due to several inherent benefits:
- Effective Decision-Making tool: MOO brings to the fore multiple conflicting objectives, allowing for the exploration of various solutions. This encourages rational decision-making based on informed trade-offs.
- Versatility: Because of its aptitude to handle multiple objectives, MOO methodology cuts across various disciplines like bioinformatics, operations research, engineering design, transportation and logistics, among others.
- Flexibility of Solutions: MOO's major advantage lies in offering multiple Pareto-optimal solutions, affording the decision-maker flexibility in their final choice. They can align their final decision with organizational strategies and policy priorities.
- Efficiency: MOO techniques can help identify redundant objectives and emphasize critical ones, thus fostering efficiency.
- Practicality: MOO enhances the feasibility of AI models by focusing on real-world problem-solving that often encompasses multiple objectives.
- Harmonizing conflicting objectives: With its suite of solutions, MOO helps harmonize goals that would otherwise conflict in a single-objective setup.
Despite its numerous benefits, MOO does have several challenges and pitfalls that must be considered.
Disadvantages of Multi-objective Optimization in AI
Decision-Makers need to consider MOO's potential downsides:
- Computationally intensive: The search for multiple optimal solutions might require substantial computational power and time, especially for problems with many objectives.
- Requires Expertise: Selecting the appropriate MOO method and effectively interpreting its results demand expert knowledge.
- Complexity: Dealing with multiple objectives adds an extra layer of complexity to the problem-solving process.
- Choice Paralysis: Having several optimal solutions brings its own dilemma—how to choose the best one.
- Risk of Ignoring Key Objectives: There's a risk of undermining essential objectives while ardently pursuing Pareto optimality.
Take Action
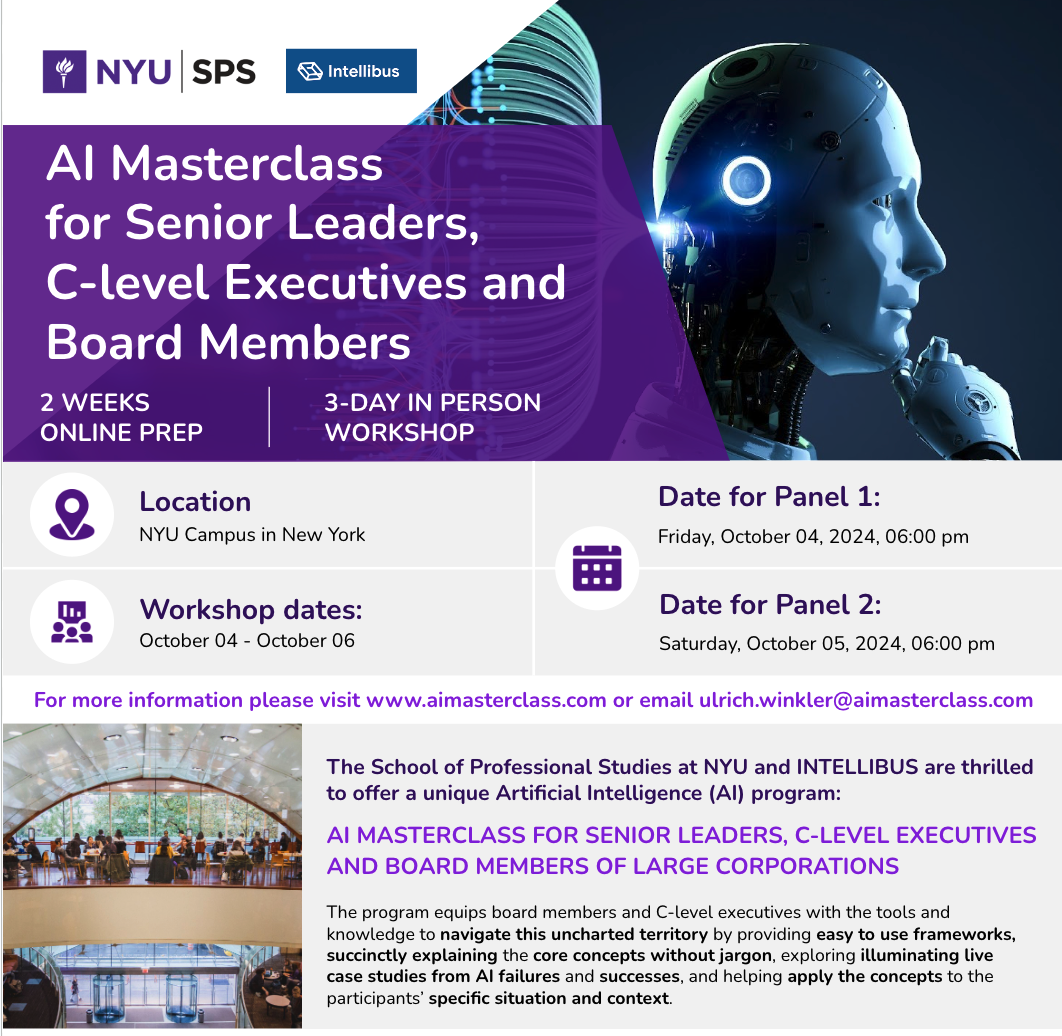
Download Brochure
- Course overview
- Learning journey
- Learning methodology
- Faculty
- Panel members
- Benefits of the program to you and your organization
- Admissions
- Schedule and tuition
- Location and logistics