What is Multimodal Learning Algorithms?
Multimodal Learning Algorithms: An Overview
Multimodal Learning Algorithms (MLA) are innovative algorithms that learn from multiple data types simultaneously, with the primary goal of improving learning performance. They take a step beyond traditional machine learning, which leverages single data types, by using diverse data sources to provide a more accurate and comprehensive model of the real world.
Key Characteristics of Multimodal Learning Algorithms:
- Broad Data Inputs: Unlike traditional algorithms, MLAs can handle diverse data types, from text and images to audios and videos. This is due to the algorithms’ ability to integrate multiple data modalities into a unified learning framework.
- Complexity Handling: MLAs are known for their ability to handle complex and large-scale data effectively, which is increasingly becoming crucial in the age of big data.
- Improved Prediction Accuracy: Incorporating multiple types of data improves the accuracy of resultant predictions and provides a more complete understanding of the phenomena under study.
- Algorithm Customization: To address the distinctive needs of different applications, MLAs often require algorithm customization to optimize data analysis and obtain accurate results.
- Task Versatility: MLAs can solve a wide range of tasks, such as image recognition, language processing, and object detection, allowing for broad industry application.
Understanding and Implementing Multimodal Learning Algorithms
Implementing MLAs necessitates a comprehensive analysis of organizational needs, precise selection of the suitable algorithm, and persistent monitoring of algorithm performance. During the implementation, an in-depth understanding of the data to be processed helps to customize the algorithm effectively. An open dialogue with data scientists and IT teams is essential to ensure effective MLA implementation and integration into existing systems. Critical evaluation of performance and continuous adaptation to the evolving needs of the business can assure the successful deployment of Multimodal Learning Algorithms.
Artificial Intelligence Master Class
Exponential Opportunities. Existential Risks. Master the AI-Driven Future.
Advantages of Multimodal Learning Algorithms
- Unmatched Data Analysis: Taking advantage of MLAs' ability to handle varied data types, organizations can dive deeper into data analytics, fostering more precise decision-making processes. MLAs benefit from the diverse data inputs, increasing their robustness and accuracy.
- Improved Accuracy: By exploiting correlations in different types of data, MLAs achieve more accurate and reliable results compared with unimodal approaches. They leverage complementary and redundant information from multiple sources to enhance prediction robustness.
- Flexibility: The adaptability of MLAs allows users to tailor their learning and prediction tasks by including varying types and quantities of data, depending on the problem at hand.
- Metamorphic Insights: MLAs can generate new insights from the interaction of different data types, providing an additional layer of depth beyond the insights each data type could offer on its own.
Disadvantages of Multimodal Learning Algorithms
- Complexity: Implementing MLAs requires a deep understanding of different data types and algorithm customization, which can introduce significant complexity.
- Data Overwhelm: While diversity in input is a significant strength for MLAs, it can also overwhelm an incorrectly configured MLA, negatively impacting its ability to make accurate predictions.
- Security Concerns: As MLAs manage larger and multifaceted datasets, they might attract potential security threats due to increased data vulnerability.
- Implementation Challenges: The process of integrating MLAs into existing workflows can be challenging due to their nature and complexity.
In conclusion, although MLAs come with their set of challenges, the advantages they offer, particularly in terms of enhanced prediction accuracy and versatility, make them an exciting prospect in the world of machine learning. Implementing them successfully, however, requires a comprehensive understanding of these algorithms and the ability to manage and process diverse data types.
Take Action
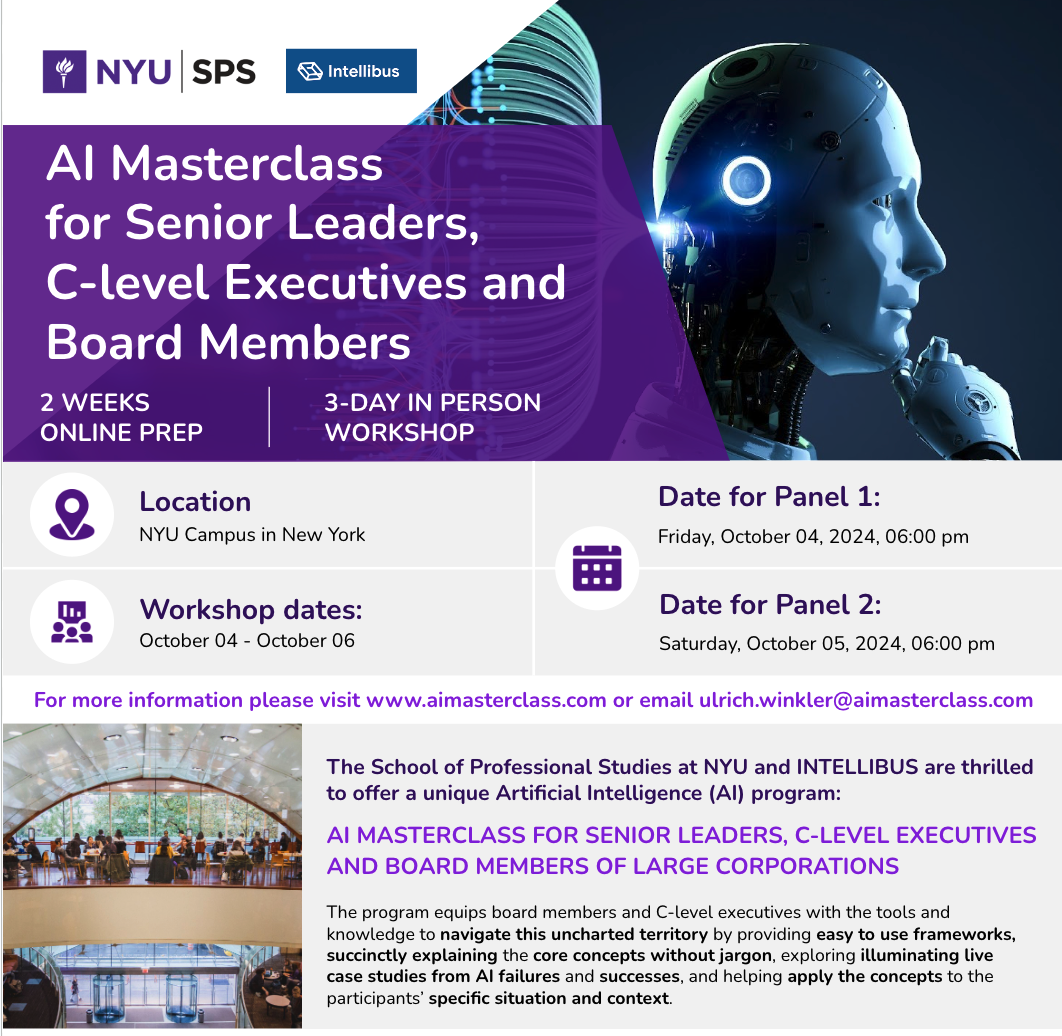
Download Brochure
- Course overview
- Learning journey
- Learning methodology
- Faculty
- Panel members
- Benefits of the program to you and your organization
- Admissions
- Schedule and tuition
- Location and logistics