What is Self-Supervised Learning for Image Classification?
What is Self-Supervised Learning for Image Classification?
Self-Supervised Learning, a relatively new paradigm in machine learning, aims to utilize large amounts of unlabeled data effectively to train machine learning models. This approach, particularly relevant to the field of Image Classification, seeks to generate its own context-dependent supervisory signals instead of reliance on labeled data.
Key attributes of Self-Supervised Learning for Image Classification:
Wide Application: Many industries use Self-Supervised Learning such as e-commerce, healthcare, social media and robotics to perform image classification tasks without the need for large labeled datasets. It allows quick generation of results based on the context.
Innovative Features: Through the provision of predictive learning tasks using unlabeled data, Self-Supervised Learning allows more extensive model training and thus improves image classification.
Learner Independence: As the name suggests, Self-Supervised Learning does not require labeled datasets, which dramatically reduces the dependency on data labeling.
Less Computational Resources: When compared to fully supervised learning tasks, Self-Supervised Learning requires lesser computational resources as it is capable of learning from fewer labeled examples, hence is cost-effective.
Improving Accuracy: With the increased availability of unlabeled data, the Self-Supervised Learning models are capable of learning more robust features and thus often achieving greater classification accuracy.
Leveraging Self-Supervised Learning for Image Classification is increasingly becoming common in many industries due to its ability to use unlabeled data effectively. This allows businesses to derive insights from images more rapidly, without the extensive requirement of labeled data.
Artificial Intelligence Master Class
Exponential Opportunities. Existential Risks. Master the AI-Driven Future.
Advantages of Self-Supervised Learning for Image Classification
Incorporating Self-Supervised Learning offers benefits such as:
Cost-effective: Utilizing the abundant unlabeled data for learning, Self-Supervised Learning reduces the need for expensive data labelling which can often be both labor-intensive and time-consuming.
Efficient and Fast: With the elimination of time spent on data labeling, the process becomes more efficient - enabling faster deployment of image classification models for organizational use.
Improved Accuracy: With more data to learn from, Self-Supervised Learning models can extract subtle patterns often missed by Supervised Learning models, thus resulting in improved accuracy in image classification tasks.
Scalability: As the models improve over time with the availability of more data, they can easily be scaled to accommodate larger datasets, making them a practical option for long-term applications.
Instant Access to Enhanced Features: Users get to take advantage of advanced features and functionalities without the need to continually curate labelled data.
Disadvantages of Self-Supervised Learning for Image Classification
Certain limitations come with Self-Supervised Learning:
Limited Control: The learning process does not provide the user with the level of control that Supervised Learning offers - this could result in models learning irrelevant or incorrect features.
Dependence on Data Quality: While Self-Supervised Learning utilizes unlabeled data, the model's performance can significantly depend on the quality of data used.
Integration Issues: Since Self-Supervised Learning is a relatively new paradigm in machine learning, integrating these models with existing systems could be complex and resource-intensive.
Higher Risk of Errors: There is a high possibility of the model learning irrelevant features, leading to errors in the final image classification output.
Complexity: As this is an emerging field, a lack of standardized practices and guidelines can make the implementation process difficult and complicated.
Implementation of Self-Supervised Learning for Image Classification
The implementation of Self-Supervised Learning for Image Classification begins with understanding organizational needs, available resources, and the complexity of the image classification task. This is followed by the preparation of the data that includes a collection, cleaning, and structuring. The model can be trained and refined over time with the availability of more data. The approach fosters continuous learning, thereby greatly improving the efficiency of the model. Careful planning, evaluation, and monitoring play a big role in successful Self-Supervised Learning implementation. Therefore, it is crucial to establish a systematic process and maintain close supervision.
Take Action
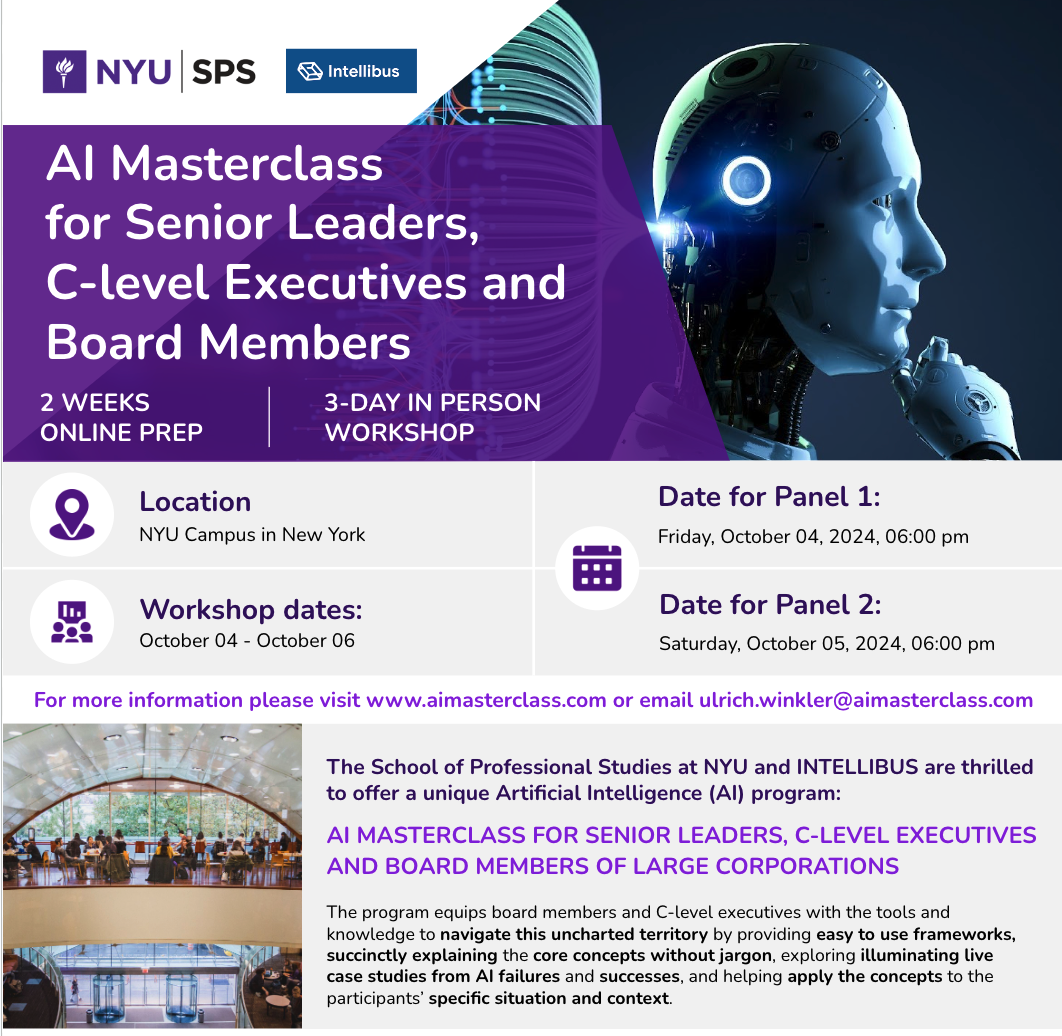
Download Brochure
- Course overview
- Learning journey
- Learning methodology
- Faculty
- Panel members
- Benefits of the program to you and your organization
- Admissions
- Schedule and tuition
- Location and logistics