What is Sentiment Classification?
Understanding Sentiment Classification
Sentiment Classification is a critical subfield in Natural Language Processing (NLP) and machine learning that involves computational methods for identifying and classifying emotions or attitudes expressed by a writer or speaker. It focuses on the extraction of subjective information in text data, seeking to comprehend sentiments, opinions, evaluations, appraisals, and emotions communicated within a text. Sentiment Classification can be both binary (positive, negative) or multiclass (positive, neutral, negative) and can be applied on several levels such as document level, sentence level or sub-sentence level.
Distinctive Principles of Sentiment Classification
- Unique Applicability: Sentiment classification applies to diverse sets of data cutting across industries and sectors, from analyzing customer reviews to gauging public sentiment on social issues. Its application is broad and versatile.
- Progressive Learning: It predominantly employs machine learning approaches which involve iterative learning, allowing the system to continuously improve and refine its knowledge base from new data.
- Complex Analysis: Sentiment analysis is not just literal interpretation, but also includes understanding linguistic nuances, sarcasm, slang, and cultural variations.
- Real-Time Processing: The majority of sentiment classifiers operate in real-time, processing real-time data feeds to deliver on-the-spot insights.
- Vendor Support: Vendors typically provide updates, customer support, and tutorials for sentiment classification tools, rendering the operation seamless.
Implementing Sentiment Classification
The successful deployment of sentiment classification solutions requires a well-articulated strategy and thorough understanding of the operational context. This includes identifying the specific application areas, understanding the nature of data being processed, and evaluating the capabilities of different vendors. Post-implementation, it is crucial to regularly evaluate the model performance and accuracy and make necessary adjustments - a process that involves continuous learning and adaptation.
As we continue to harness the power of machine learning and artificial intelligence in enhancing business processes, sentiment classification will undoubtedly play a pivotal role. Its potential to provide immediate and in-depth insights into customer attitudes and opinions equip businesses with a powerful tool in their strategic arsenal. It is, therefore, vital to understand its functioning, its potential benefits, and potential drawbacks to leverage it effectively in diverse organizational contexts.
Artificial Intelligence Master Class
Exponential Opportunities. Existential Risks. Master the AI-Driven Future.
Advantages of Sentiment Classification
The adoption of sentiment classification offers a plenitude of advantages, including:
- Cost-Effective: Sentiment Classification enables organizations to automatically analyze customer feedback or social media conversations, hence reducing the costs associated with manual data processing.
- Efficiency: Manual analysis of sentiment can be time-consuming and labor-intensive. Sentiment classification, which employs sophisticated algorithms, significantly cuts down processing time.
- Insights Generation: It can detect hidden patterns and trends, aiding businesses in gaining in-depth understanding of their customer base thereby informing strategic decisions.
- Enhanced Customer Experience: By analyzing customer sentiments, businesses can tailor their products and communication strategies to meet customer needs and enhance their experience.
- Scalability: Sentiment classification can handle large volumes of data efficiently, eliminating the limits of manual analysis, and hence offering scalability.
However, as with any technology, sentiment classification presents its own set of challenges.
Challenges in Sentiment Classification
While it offers many advantages, organizations must keenly consider certain pitfalls:
- Linguistic Variance: The complexity of human language, including usage of sarcasm, irony, region-specific dialects, or cultural variations, can pose significant challenges to accurate sentiment analysis.
- Limited Influence: Users usually lack control over updates and enhancements of sentiment classification tools provided by vendors, which may lead to discrepancies with specific organizational needs.
- Integration Hurdles: The scalability and efficiency of deployed sentiment classifiers highly depend on their integration with existing systems.
- Security Concerns: Machine learning models utilized in sentiment analysis can be targets of data poisoning attacks where maliciously biased training data is used to skew the model.
Take Action
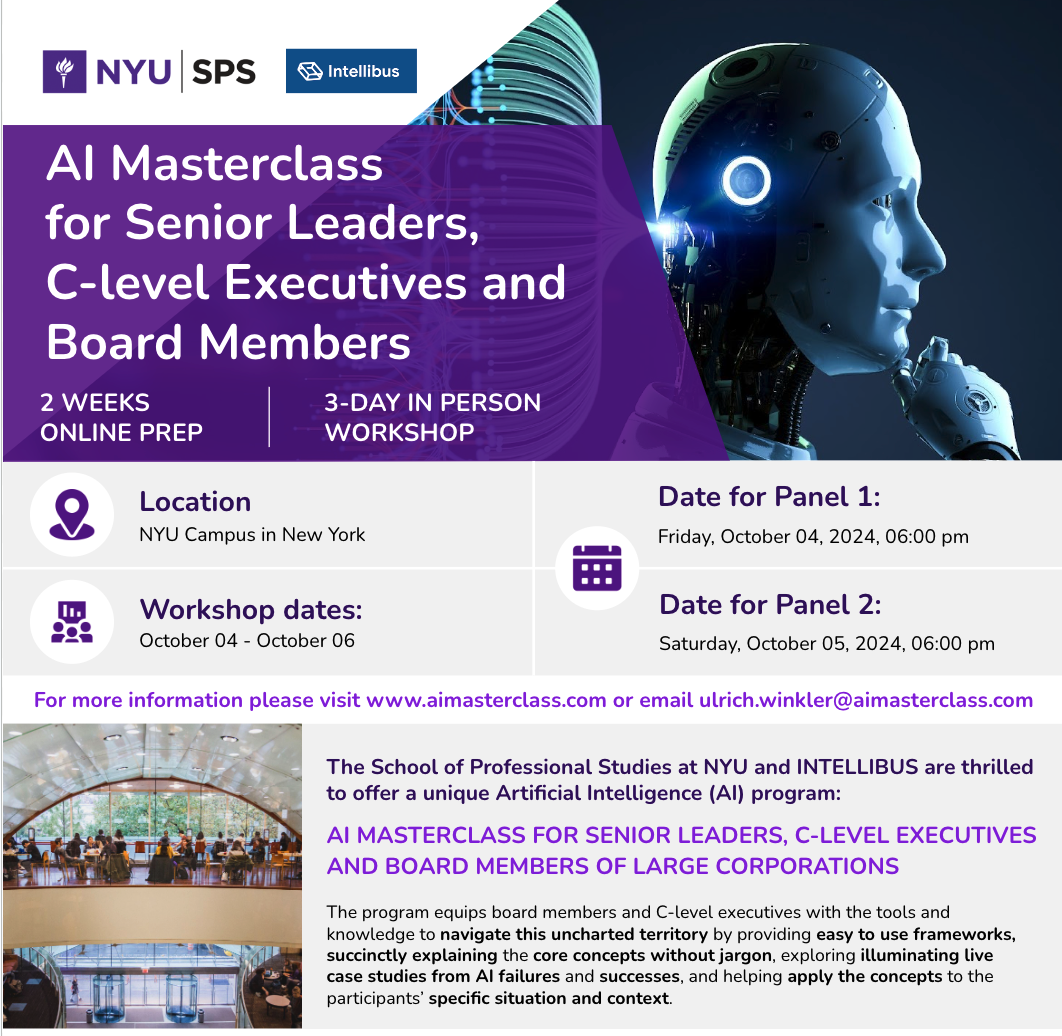
Download Brochure
- Course overview
- Learning journey
- Learning methodology
- Faculty
- Panel members
- Benefits of the program to you and your organization
- Admissions
- Schedule and tuition
- Location and logistics