What is Social Network Analysis for Community Detection?
Social Network Analysis (SNA) for Community Detection
Social Network Analysis for Community Detection, often abbreviated as SNA, is a specialized approach applied across various scientific fields such as data analysis, sociology, and computer science, among others, to reveal and understand the structure and patterns of relationships within a given population or community.
SNA applies mathematical theories and algorithms to map, measure and investigate complex networks or systems. This technique is invaluable when identifying and clustering smaller communities within large, dynamic networks, improving how we visualize and understand the structure and behaviors within these networks.
Key Characteristics of SNA for Community Detection:
Broad Application: The broad utility of SNA makes it a versatile tool applicable across multiple disciplines – from social sciences and biology to computer networks and business intelligence, among others.
Complex Structure Analysis: Through SNA, analysts can unravel and understand the often complex structures within networks, mapping out relationships and interactions.
Community Detection: SNA is often used to detect communities within larger, complex networks, by identifying closely grouped nodes that regularly interact with each other, but having fewer interactions with nodes outside the group.
- Actionable Insights: SNA provides valuable insights that can guide decision-making processes, strategy formulation, or policy direction within an organization or social setting.
Implementation and Use of SNA for Community Detection
Effective use of SNA calls for proper planning and understanding of the specific goals you aim to achieve. The initial stages involve defining the boundaries of the network to analyze and identifying the types of relationships or interactions to focus on.
The next step is data gathering. This could involve surveys, observation, or extraction of existing data from databases or other digital platforms. After data collection comes the analysis, using specialized software tools designed for SNA.
The data can then be visualized in forms that allow for easy understanding and interpretation of the network structure and interactive patterns. With these insights drawn from the SNA, decisions can be made or policies directed to respond appropriately to the realities of the community existing within the network. Thus, the effectiveness of SNA, nonetheless, hinges on careful planning, precise data collection, a suitable choice of SNA methods, and insightful interpretation of the results.
Artificial Intelligence Master Class
Exponential Opportunities. Existential Risks. Master the AI-Driven Future.
Advantages of Using SNA for Community Detection
Precise Community Identification: SNA allows for the precision in identifying smaller, interactively cohesive groups within larger networks. This precision aids in understanding the nuanced dynamics within a community.
Informed Decision-making: The insights gathered through SNA provide valuable information and direction for decision-making, enhancing strategic foresight in business planning, policy formulation, or response strategies.
Efficient Resource Allocation: By identifying distinct communities within larger networks, resource allocation can be done with efficiency, targeting specific groups based on their unique characteristics and needs.
Identification of Key Players: SNA methods enable the identification of key nodes (individuals or groups). These are actors that hold influence within the network through their numerous or strategic connections.
Dynamic Evolution Tracking: SNA can track the evolution and changes within a network over time, providing insights into how the structure, relationships, or influence within a network changes.
Disadvantages of Using SNA for Community Detection
Complexity: As SNA tackles the analysis of complex network systems, it can be laborious and requires a substantial understanding of both the subject matter and mathematical algorithms used.
Privacy Concerns: SNA involves analysis of individuals and their interactions within a network, which can potentially pose privacy concerns, especially if adequate measures are not put in place to protect personal data.
Data Quality and Accuracy: The effectiveness of SNA relies heavily on the quality and accuracy of the data analyzed. Inaccurate or poor-quality data could lead to misleading analysis and results.
Take Action
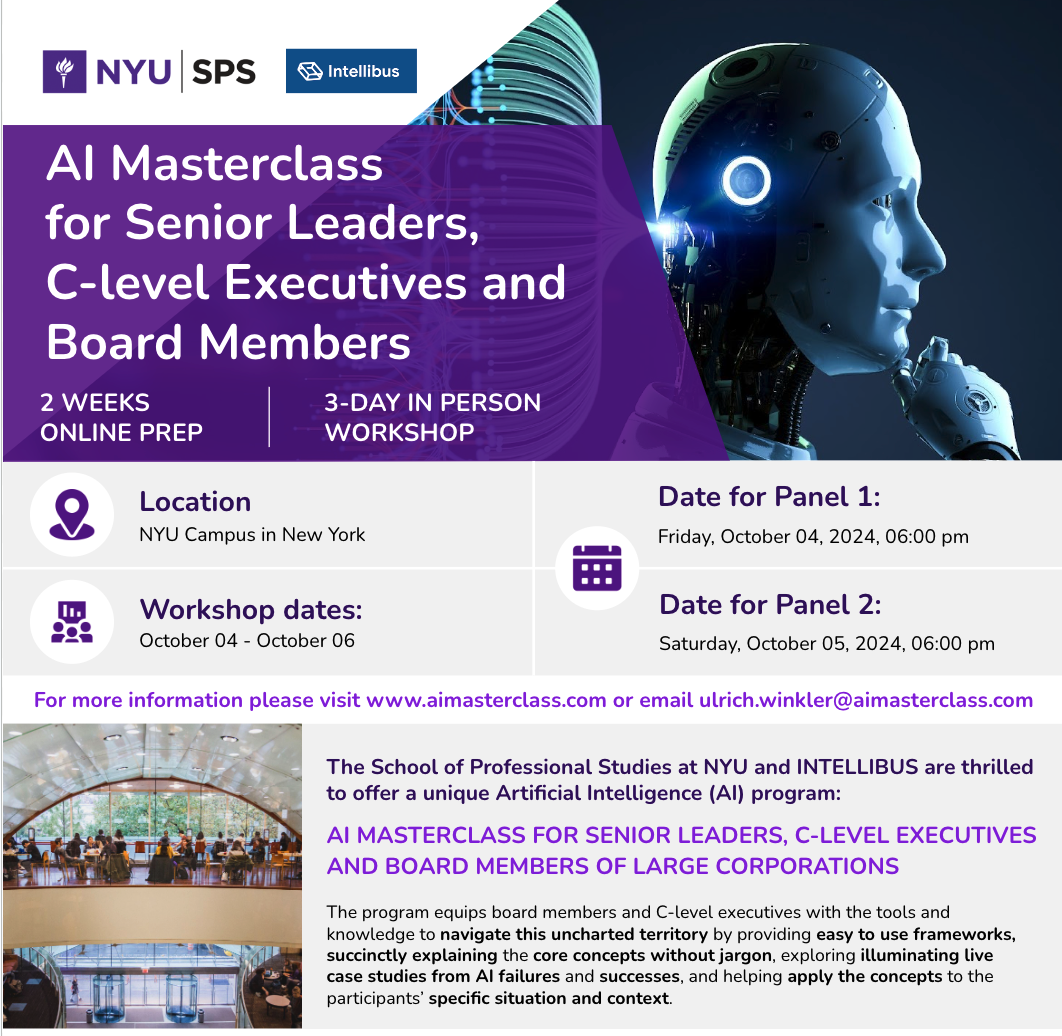
Download Brochure
- Course overview
- Learning journey
- Learning methodology
- Faculty
- Panel members
- Benefits of the program to you and your organization
- Admissions
- Schedule and tuition
- Location and logistics