What is Spiking Neural Networks?
What exactly are Spiking Neural Networks?
Spiking Neural Networks, often referred to as SNNs, stand as third-generation neural network models. Drawing their design inspiration from natural, biological nervous systems, these networks take advantage of the fact that neurons in the human brain communicate through spikes.
Central features of Spiking Neural Networks:
Temporal Computing: A cornerstone of SNNs, temporal computing allows the network to process data time-series using significantly less computational resources than traditional methods.
Biologically Inspired: Resembling the human brain's operating mechanisms, SNNs exhibit spikes or 'fireings' during computing. This makes them a powerful tool for applications such as robotics, where modeling human-like responses is valuable.
Neuroplasticity: The SNN's innovative model allows for neuroplasticity, the ability to adjust and grow based on experiences. This striking capability means the network can learn and adapt over time.
Energy Efficiency: As they mimic the brain's neuron behavior, SNNs are more energy-efficient compared to other artificial networks. This is especially useful in application areas with energy constraints, such as embedded systems or IoT devices.
Utilization of Latency: SNNs use latency as an information carrier, a feature that is often ignored by other models. This unique characteristic allows information processing in a more compact and efficient way.
Implementation of Spiking Neural Networks
Deploying SNNs necessitates careful planning and understanding of the system at hand. This begins with establishing the precise requirements set forth by the task to be accomplished. The next step involves choosing a suitable SNN architecture that aligns with the task's specifications and restrictions.
Subsequently, the SNN model needs to be trained using relevant data, prepared for the specific task in question. Model optimization is crucial to ensure the SNN operates at the best performance level. Once implemented, the SNN requires regular evaluation to maintain its performance and contribute meaningfully to the application it is supporting.
The central point is that while the adoption of Spiking Neural Networks comes with its own set of challenges, the potential rewards in terms of power, efficiency, and versatility are considerable. By bridging the gap between computation and neuroscience, these innovative models herald a promising future for AI, neural computation, and robotics. To maximize their potential, continuous refinement and advancement are essential. Enhanced understanding, improved tools, and refined techniques will undoubtedly bring forth a new era in AI, driven by SNNs.
Artificial Intelligence Master Class
Exponential Opportunities. Existential Risks. Master the AI-Driven Future.
Advantages of Spiking Neural Networks
Increased Efficiency: As SNNs operate with spikes that save computational resources, they're considerably more energy-efficient. This efficiency comes without sacrificing model accuracy, providing excellent performance levels across various applications.
Temporal Precision: SNNs can process time-series data while preserving the temporal accuracy of input signals. This is beneficial in applications where timing information is crucial, like audio and video processing, event prediction, and others.
Versatility: SNNs are compatible with a vast range of applications. From robotics and AI to IoT and neuromorphic hardware, Spiking Neural Networks can effectively tackle numerous tasks.
- Learning Potential: Thanks to their neuroplasticity, SNNs model continuous learning effectively. This on-the-fly learning capability helps overcome the static training and deployment flow of traditional artificial neural networks.
Disadvantages of Spiking Neural Networks
Complexity: Because of the advanced mechanisms involved, understanding and programming Spiking Neural Networks can be complex. This can serve as a potential hurdle for their mainstream adoption.
Availability of Tools: The computational neuroscience field still lacks an assortment of tools for SNNs, potentially slowing their proliferation in the artificial intelligence (AI) industry.
Computational resources: Although SNNs are generally efficient, large SNNs can demand extensive computational resources. Capabilities such as neuroplasticity sometimes come at the cost of increased computational requirements.
- Limited Influence: The amount of control one can exert over the learning process within SNNs isn’t as straightforward as in traditional artificial neural networks, which can be a limitation for some applications.
Take Action
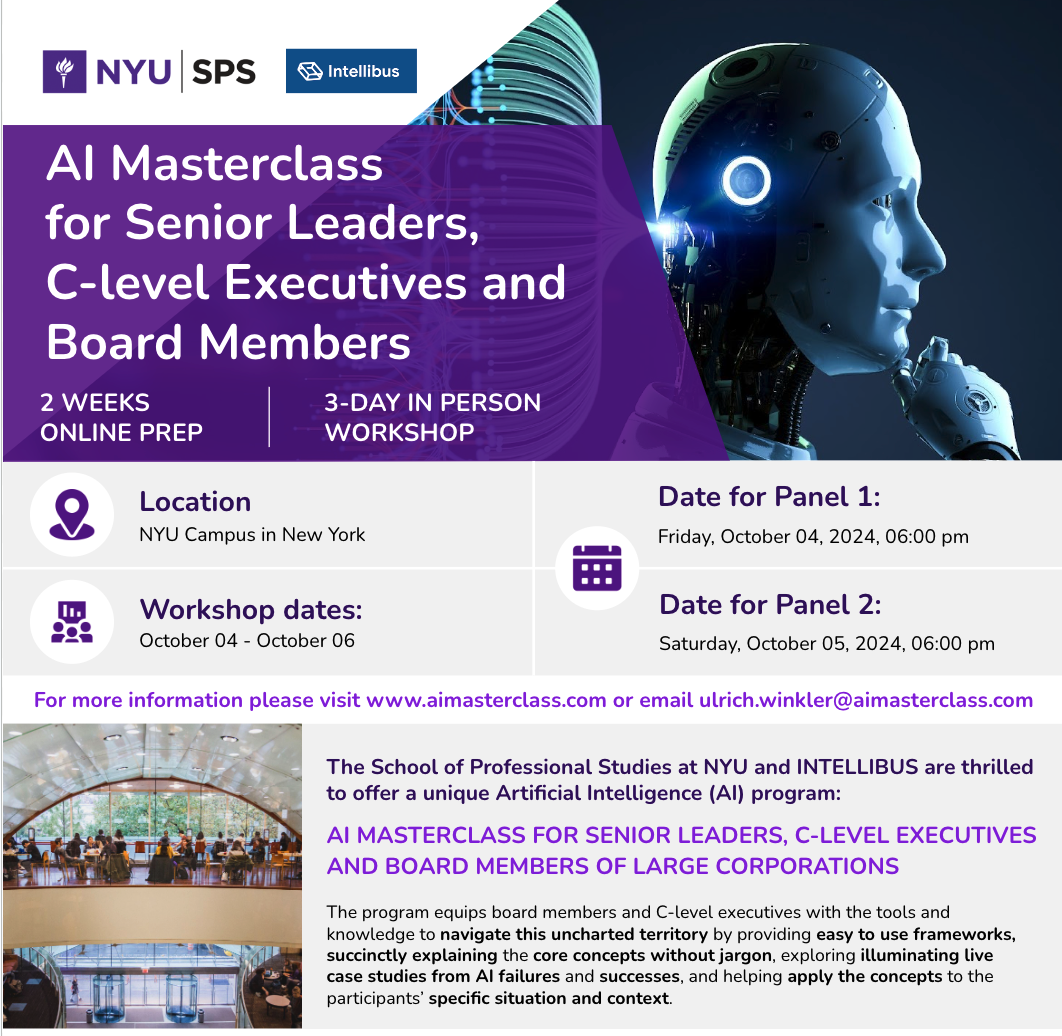
Download Brochure
- Course overview
- Learning journey
- Learning methodology
- Faculty
- Panel members
- Benefits of the program to you and your organization
- Admissions
- Schedule and tuition
- Location and logistics